Fixing among the main challenges of the twenty first Century, similar to producing clear electrical energy or growing excessive temperature superconductors, would require us to design new supplies with particular properties. To do that on a pc requires the simulation of electrons, the subatomic particles that govern how atoms bond to kind molecules and are additionally answerable for the circulate of electrical energy in solids. Regardless of a long time of effort and a number of other important advances, precisely modelling the quantum mechanical behaviour of electrons stays an open problem. Now, in a paper (Open Access PDF) printed in Science, we suggest DM21, a neural community attaining cutting-edge accuracy on massive elements of chemistry. To speed up scientific progress, we’re additionally open sourcing our code for anybody to make use of.
Almost a century in the past, Erwin Schrödinger proposed his famous equation governing the behaviour of quantum mechanical particles. Making use of this equation to electrons in molecules is difficult as a result of all electrons repel one another. This would appear to require monitoring the likelihood of every electron’s place — a remarkably advanced process for even a small variety of electrons. One main breakthrough got here within the Nineteen Sixties, when Pierre Hohenberg and Walter Kohn realised that it’s not mandatory to trace every electron individually. As a substitute, realizing the likelihood for any electron to be at every place (i.e., the electron density) is enough to precisely compute all interactions. Kohn obtained a Nobel Prize in Chemistry after proving this, thus founding Density Useful Concept (DFT).
Though DFT proves a mapping exists, for greater than 50 years the precise nature of this mapping between electron density and interplay power — the so-called density purposeful — has remained unknown and must be approximated. Although DFT intrinsically includes a degree of approximation, it’s the solely sensible technique to review how and why matter behaves in a sure manner on the microscopic degree and has due to this fact turn into one of the vital broadly used strategies in all of science. Over time, researchers have proposed many approximations to the precise purposeful with various ranges of accuracy. Regardless of their reputation, all of those approximations endure from systematic errors as a result of they fail to seize sure essential mathematical properties of the precise purposeful.
By expressing the purposeful as a neural community and incorporating these precise properties into the coaching knowledge, we study functionals free from necessary systematic errors — leading to a greater description of a broad class of chemical reactions.
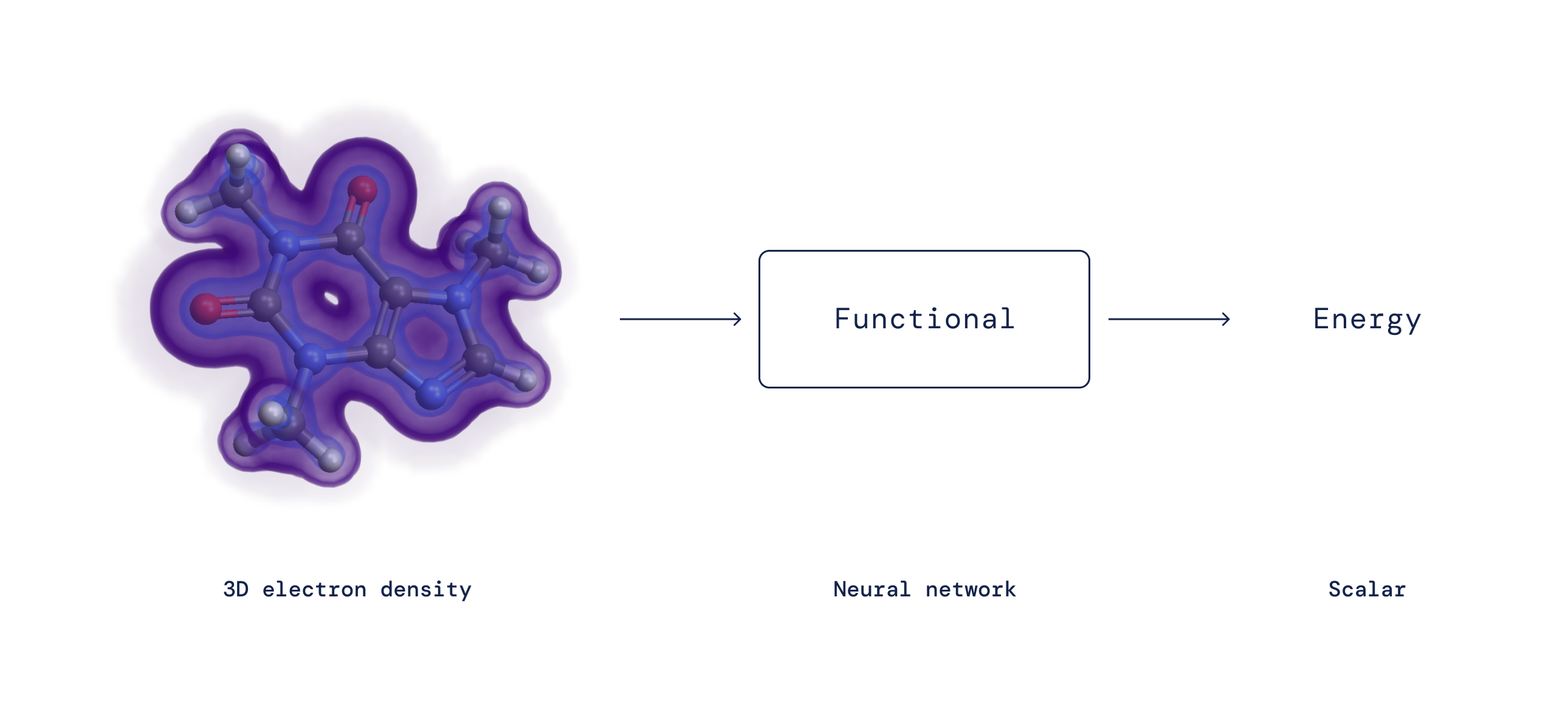
We particularly tackle two long-standing issues with conventional functionals:
- The delocalization error: In a DFT calculation, the purposeful determines the cost density of a molecule by discovering the configuration of electrons which minimizes power. Thus, errors within the purposeful can result in errors within the calculated electron density. Most current density purposeful approximations favor electron densities which might be unrealistically unfold out over a number of atoms or molecules somewhat than being accurately localized round a single molecule or atom (see Fig 2).
- Spin symmetry breaking: When describing the breaking of chemical bonds, current functionals are likely to unrealistically favor configurations by which a elementary symmetry generally known as spin symmetry is damaged. Since symmetries play a significant position in our understanding of physics and chemistry, this synthetic symmetry breaking reveals a serious deficiency in current functionals.
In precept, any chemical-physical course of that includes motion of cost is liable to endure from delocalization error, and any course of that includes the breaking of bonds is liable to endure from spin-symmetry breaking. Motion of cost and bond breaking are core to many necessary technological functions, however these issues can even result in large qualitative failure of functionals to explain the only molecules, similar to hydrogen. Since DFT is such an important expertise you will need to design functionals that get this easy chemistry right earlier than asking them to clarify vastly extra advanced molecular interactions, similar to those who might happen in a battery or photo voltaic cell.
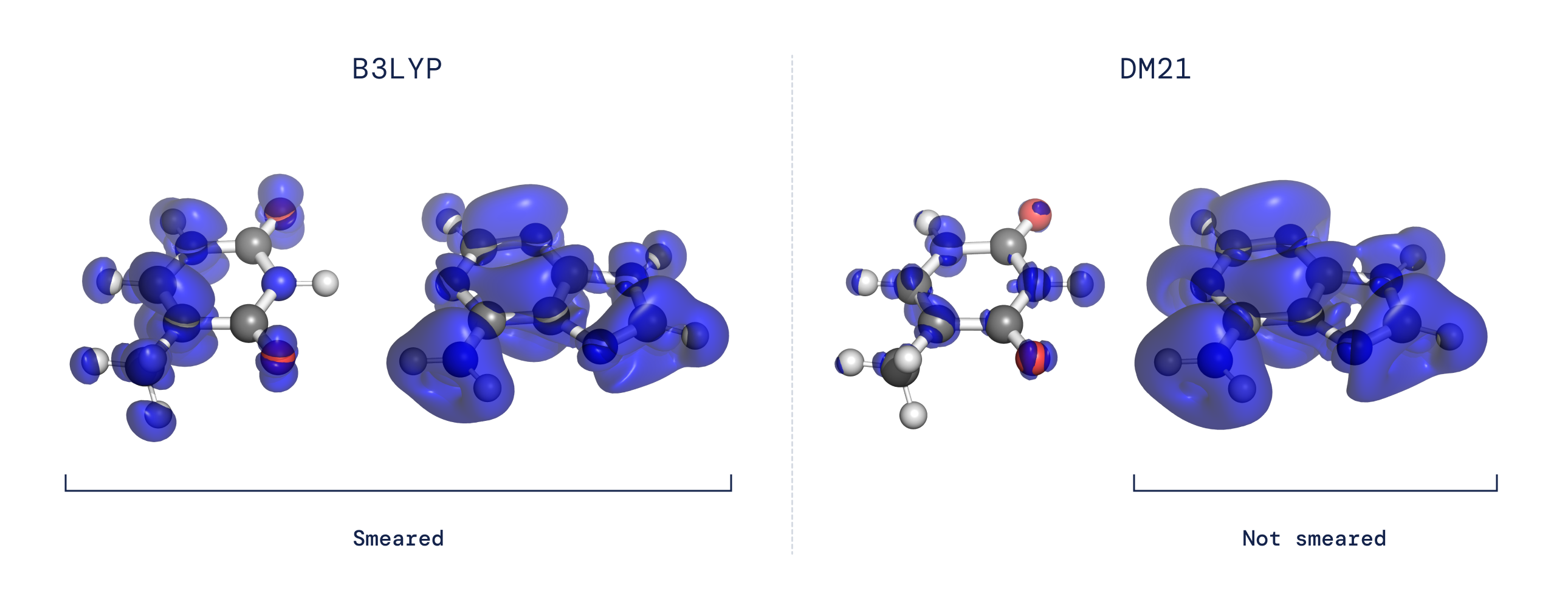
These longstanding challenges are each associated to how functionals behave when introduced with a system that displays “fractional electron character.” Through the use of a neural community to characterize the purposeful and tailoring our coaching dataset to seize the fractional electron behaviour anticipated for the precise purposeful, we discovered that we may clear up the issues of delocalization and spin symmetry-breaking. Our purposeful additionally confirmed itself to be extremely correct on broad, large-scale benchmarks, suggesting that this data-driven strategy can seize elements of the precise purposeful which have up to now been elusive.
For years, laptop simulations have performed a central position in trendy engineering, making it doable to supply dependable solutions to questions like “will this bridge keep up?” to “will this rocket make it into house?” As expertise more and more turns to the quantum scale to discover questions on supplies, medicines, and catalysts, together with these we’ve by no means seen and even imagined, deep studying exhibits promise to precisely simulate matter at this quantum mechanical degree.