To coach brokers to work together nicely with people, we’d like to have the ability to measure progress. However human interplay is advanced and measuring progress is tough. On this work we developed a way, known as the Standardised Take a look at Suite (STS), for evaluating brokers in temporally prolonged, multi-modal interactions. We examined interactions that encompass human members asking brokers to carry out duties and reply questions in a 3D simulated surroundings.
The STS methodology locations brokers in a set of behavioural situations mined from actual human interplay information. Brokers see a replayed situation context, obtain an instruction, and are then given management to finish the interplay offline. These agent continuations are recorded after which despatched to human raters to annotate as success or failure. Brokers are then ranked in response to the proportion of situations on which they succeed.
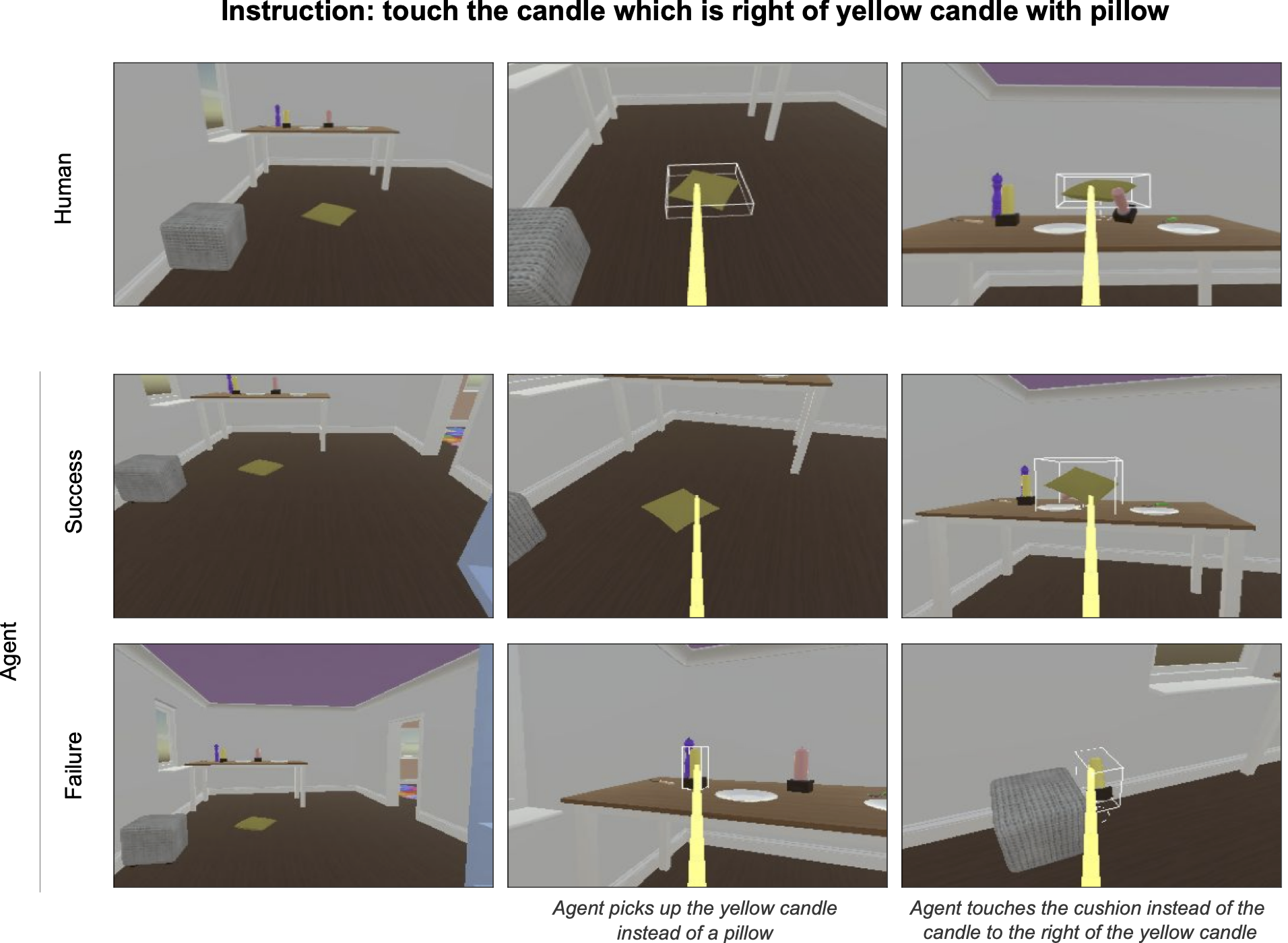
Lots of the behaviours which are second nature to people in our day-to-day interactions are tough to place into phrases, and unimaginable to formalise. Thus, the mechanism relied on for fixing video games (like Atari, Go, DotA, and Starcraft) with reinforcement studying will not work once we attempt to educate brokers to have fluid and profitable interactions with people. For instance, take into consideration the distinction between these two questions: “Who received this sport of Go?” versus “What are you ?” Within the first case, we are able to write a chunk of laptop code that counts the stones on the board on the finish of the sport and determines the winner with certainty. Within the second case, we don’t know find out how to codify this: the reply might rely upon the audio system, the dimensions and shapes of the objects concerned, whether or not the speaker is joking, and different features of the context through which the utterance is given. People intuitively perceive the myriad of related elements concerned in answering this seemingly mundane query.
Interactive analysis by human members can function a touchstone for understanding agent efficiency, however that is noisy and costly. It’s tough to manage the precise directions that people give to brokers when interacting with them for analysis. This type of analysis can also be in real-time, so it’s too gradual to depend on for swift progress. Earlier works have relied on proxies to interactive analysis. Proxies, comparable to losses and scripted probe duties (e.g. “raise the x” the place x is randomly chosen from the surroundings and the success perform is painstakingly hand-crafted), are helpful for gaining perception into brokers shortly, however don’t truly correlate that nicely with interactive analysis. Our new methodology has benefits, primarily affording management and pace to a metric that carefully aligns with our final objective – to create brokers that work together nicely with people.
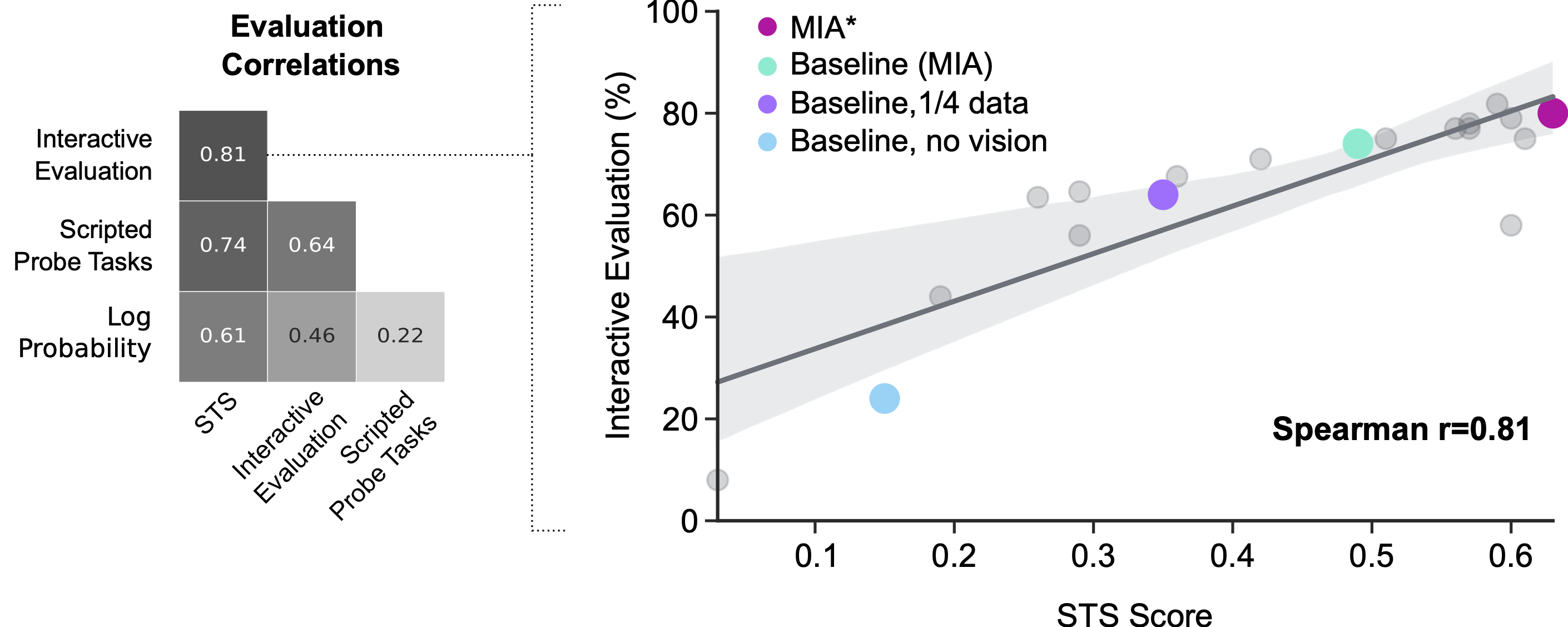
The event of MNIST, ImageNet and different human-annotated datasets has been important for progress in machine studying. These datasets have allowed researchers to coach and consider classification fashions for a one-time price of human inputs. The STS methodology goals to do the identical for human-agent interplay analysis. This analysis methodology nonetheless requires people to annotate agent continuations; nevertheless, early experiments counsel that automation of those annotations could also be doable, which might allow quick and efficient automated analysis of interactive brokers. Within the meantime, we hope that different researchers can use the methodology and system design to speed up their very own analysis on this space.