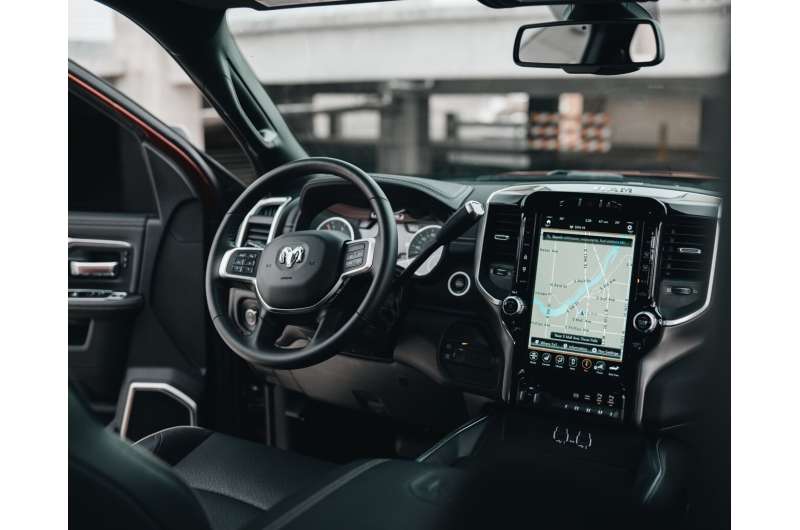
In a promising improvement for self-driving automotive expertise, a analysis workforce at NYU Tandon College of Engineering has unveiled an algorithm—often called Neurosymbolic Meta-Reinforcement Lookahead Studying (NUMERLA)—that might handle the long-standing problem of adapting to unpredictable real-world eventualities whereas sustaining security.
The analysis was performed by Quanyan Zhu, NYU Tandon affiliate professor {of electrical} and laptop engineering, and his Ph.D. candidate Haozhe Lei. It seems on the pre-print server arXiv.
Synthetic intelligence and machine studying have helped self-driving vehicles function in more and more intricate eventualities, permitting them to course of huge quantities of knowledge from sensors, make sense of advanced environments, and navigate metropolis streets whereas adhering to visitors guidelines.
As they enterprise past managed environments into the chaos of real-world visitors, nevertheless, such automobiles’ efficiency can falter, probably resulting in accidents.
NUMERLA goals to bridge the hole between security and flexibility. The algorithm achieves this by constantly updating security constraints in real-time, guaranteeing that self-driving vehicles can navigate unfamiliar eventualities whereas sustaining security as the highest precedence.
The NUMERLA framework operates as follows: When a self-driving automotive encounters an evolving surroundings, it makes use of observations to regulate its “perception” concerning the present scenario. Primarily based on this perception, it makes predictions about its future efficiency inside a specified timeframe. It then searches for acceptable security constraints and updates its information base accordingly.
The automotive’s coverage is adjusted utilizing lookahead optimization with security constraints, leading to a suboptimal however empirically protected on-line management technique.
One of many key improvements of NUMERLA lies in its lookahead symbolic constraints. By making conjectures about its future mode and incorporating symbolic security constraints, the self-driving automotive can adapt to new conditions on the fly whereas nonetheless prioritizing security.
The researchers examined NUMERLA in a pc platform that simulates city environments—particularly to establish its capability to accommodate jaywalkers—and it outperformed different algorithms in these eventualities.
Extra data:
Haozhe Lei et al, Neurosymbolic Meta-Reinforcement Lookahead Studying Achieves Secure Self-Driving in Non-Stationary Environments, arXiv (2023). DOI: 10.48550/arxiv.2309.02328
arXiv
NYU Tandon College of Engineering
Quotation:
Researchers develop algorithm for safer self-driving vehicles (2023, September 15)
retrieved 15 September 2023
from
This doc is topic to copyright. Aside from any honest dealing for the aim of personal examine or analysis, no
half could also be reproduced with out the written permission. The content material is supplied for data functions solely.