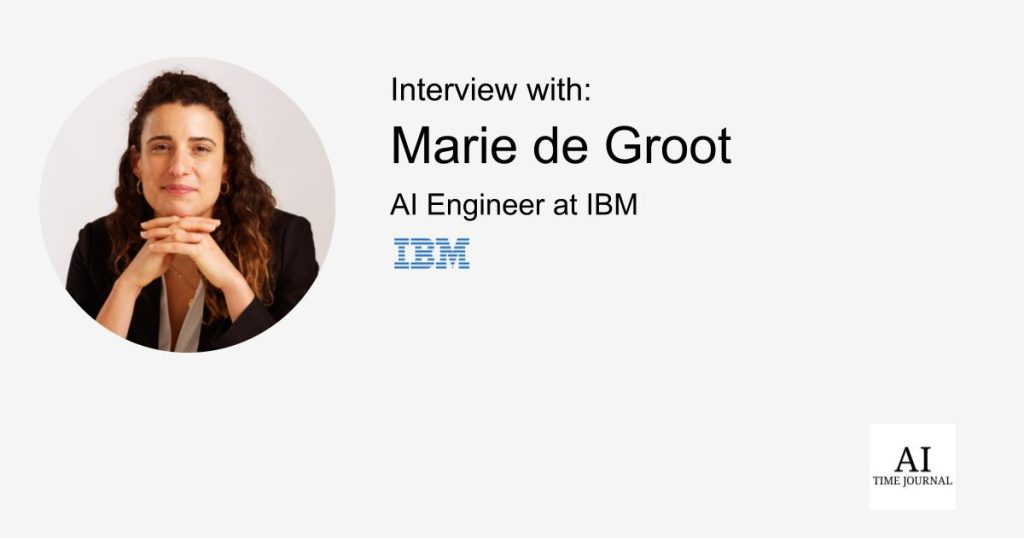
As an AI Engineer at IBM with dual Master’s degrees, Marie de Groot has accumulated five years of experience working with start-ups and multinational corporations. She is recognized for her expertise in developing and refining Generative AI and Machine Learning models for major banks and telecommunications companies. As a public speaker and the Chair of the IBM Youth Board, she is dedicated to promoting AI-led digital transformation. Her areas of expertise include AI governance, generative AI, and machine learning. She is passionate about discussing the impact of AI and automation on business, generative AI pilots, and MVPs.
Can you share your journey from earning two Master’s degrees to becoming an AI Engineer at IBM? What key experiences have shaped your career path?
My Master’s in Industrial Design Engineering in the Netherlands was a creative whirlwind— I spent most my time sketching ideas on post-its, brainstorming solutions, and refining designs. I enjoyed it but was eager to get a deeper understanding of technology. I turned to online tutorials and taught myself programming languages like HTML, JavaScript and Python to learn how to build things.
This opened doors to new possibilities that would later shape my career.
During my time in Delft, I wrote my dissertation on AI, which ignited my interest in the field. The project allowed me to delve into the potential of AI shaping industries. However, as graduation approached, I realized that while my peers were heading into traditional roles in construction and manufacturing, I craved a broader impact. I didn’t want to spend my career programming the foundations of buildings or products. AI became my vision.
To broaden my horizons, I pursued a second Master’s in business in Edinburgh. Again, centering my dissertation around AI. But studying in the UK came with a hefty price tag. So, I relied on my programming skills to start a web development side hustle, working tirelessly and saving every penny for my tuition for Edinburgh. The move to the UK opened my eyes to London. A city buzzing with innovation and cultural richness. Its energy matched my ambitions perfectly. It was during this time that I found my home at IBM in London, where I currently work as an AI Engineer. At IBM, I create AI solutions for banking and telecom. From initial ideas to practical applications, my role blends technical know-how with understanding business needs.
Reflecting on my journey, I encourage students nearing graduation to never feel confined by the path you started on. University isn’t about following a predetermined path; it’s about exploring new skills and passions. You can write your own story! You can, quite literally, shape your future and achieve your dreams and pursue what truly excites you. My journey from designer, to self-taught programmer to AI Engineer has shown me that with determination, continuous learning, and a willingness to take risks, you can forge your own path and achieve your dreams. Don’t be afraid to step out of your comfort zone—it’s where the most rewarding experiences and opportunities often lie.
As the founder and an AI Engineer with experience in both start-ups and multinationals, how do you see the differences in AI adoption and implementation between these two types of organizations?
Absolutely. Traditionally, large enterprises have enjoyed a significant advantage in AI adoption.
In the past, organizations only used traditional AI models. If you wanted to use AI, it would be a significant investment decision. You had to form teams of experts, process large amounts of data by labelling it, and work together for months. Each AI application was only suited for one specific use case. If you wanted to use AI for another use case, you had to start all over again, making it a lengthy and resource-intensive process. This situation favored large enterprises that could afford such investments, while startups typically couldn’t due to their limited resources.
However, the advent of large language models has fundamentally changed the dynamics of how businesses adopt AI. These models use large neural networks trained extensively on unlabeled data through self-supervised learning, dramatically reducing the human effort required for data ingestion. This capability allows for the efficient processing of vast amounts of data with minimal human intervention. And if you can (relatively) easily ingest lots and lots of data, of course the model becomes superior in tasks such as text generation and image processing. Almost as good as how a human would do it/mimicking human behavior.
Moreover, these “base” or foundational models can be applied across multiple downstream tasks. Organizations can now leverage pre-trained models directly, without the need for extensive initial investment or starting from scratch for each application. This streamlined approach enables rapid deployment of AI solutions, reducing deployment times from months to mere seconds, without needing any data. Consequently, these advancements have levelled the playing field for both multinationals and startups. Organizations of all sizes can now harness the power of AI swiftly and effectively, unlocking new possibilities without the previous barriers to entry.
So how do they adopt AI differently?
In my experience, I’ve observed distinct approaches to AI adoption between multinationals and startups, although my observations are primarily based on my projects rather than broader research.
Multinationals, known for their risk aversion and rigorous legal scrutiny, often prioritize AI applications that reduce costs and enhance efficiency across sectors like HR, customer service, finance, marketing, sales, and IT. Chatbots, for instance, represent low-risk, high-reward opportunities due to their proven utility in these areas.
In contrast, start-ups thrive on disruptive innovation, using AI to streamline operations and drive rapid growth. With fewer resources, they focus on high-impact use cases around their product related business functions. Often resulting in different pilots than chatbots. Unlike multinationals, start-ups are less constrained by established processes and are more willing to take risks to enhance their core product offerings.
In summary, while multinationals consolidate their market dominance through cost-cutting measures and established AI applications, start-ups leverage AI to innovate rapidly and enhance their competitive edge. The levelling of entry barriers by foundation models has created a more equitable playing field, enabling both types of organizations to harness AI’s transformative potential in distinct but equally impactful ways.
Generative AI is a rapidly evolving field. Could you walk us through one of your notable projects where you successfully built and tuned a generative AI model for a major bank or telco?
Yes I worked on this project. More information can be found here
What are the common challenges that organizations face when adopting generative AI, and how do you help your clients navigate these hurdles?
When adopting generative AI, organizations commonly face several challenges that require careful navigation. One of the most pressing challenges I’ve witnessed is in testing these cutting-edge solutions. Positioned at the forefront of the generative AI market, we find ourselves with few established guidelines to follow. Many executives are venturing into uncharted territory, crafting strategies on the fly as they pioneer this transformative technology.
Clients often focus on metrics like accuracy, latency, and output quality to gauge the performance of generative AI solutions, largely influenced by recommendations online or courses. Yet, in my experience—drawn primarily from hands-on projects rather than broad research—I’ve observed that AI vendors tend to compete on a sliding scale, with marginal differences between models.
While typical pilots yield impressive results:
– Accuracy hovers around 95%.
– Latency ranges from 60 to 90 milliseconds, adjustable to meet specific needs.
– Output customization is fully adaptable to cater precisely to client requirements.
I challenge whether solely competing on these metrics aligns with the overarching goal of harnessing AI’s potential. Instead of solely pursuing incremental gains in technical performance, organizations should prioritize holistic evaluations. These assessments must encompass governance, bias mitigation, and ethical considerations. Such measures are pivotal for establishing trust in AI systems, safeguarding against misuse, and preventing scrutiny from the public and stakeholders alike. Addressing queries like access to sensitive data or maintaining ethical standards in AI deployment are key during testing phases. A simple guideline isn’t sufficient; organizations need to understand how their models perform in real-world production scenarios. Monitoring bias, fairness, and drift in production is key to ensuring the continued ethical integrity and effectiveness of AI applications.
At IBM, we steadfastly uphold the principles of trustworthy AI through initiatives like IBM watsonx governance. This framework ensures the robustness, ethical integrity, and readiness of AI systems for real-world deployment.
In essence, while metrics provide initial benchmarks, the true test of generative AI lies in upholding trustworthiness and ethical standards amidst a rapidly evolving landscape where best practices are still emerging. By integrating robust governance frameworks and proactive measures, organizations can confidently pilot and deploy generative AI solutions that meet both technical and ethical standards.
As the Chair of the IBM Youth Board, how do you see the role of young professionals in driving AI-led digital transformation?
I truly believe that young professionals are crucial in driving AI-led digital transformation. Generative AI can do so much more than just being a chatbot.
We face huge challenges like overpopulation, climate change, food insecurity, deforestation, and the exploration of space. I hope that generative AI, powered by quantum computing, will help us tackle these issues by bringing new ideas and solutions to the table.
Imagine AI systems with quantum computing capabilities, analyzing huge amounts of data at lightning speed and with great accuracy. These advancements could revolutionize climate modelling, helping us make better predictions and strategies to protect the environment. IBM is already working with NASA to tackle Amazonian deforestation by developing Hugging Face’s largest geospatial model. Generative AI could also optimize farming practices to ensure food security, simulate ecosystems to protect wildlife, and develop new energy solutions to fight resource depletion and hunger.
Plus, AI could help us in space exploration by improving spacecraft design, making it easier to analyze space data, and supporting long-term space missions. By using generative AI and quantum computing, our generation has a chance to lead innovations that not only change industries but also help solve some of the world’s biggest problems.
In short, our generation can use AI technologies responsibly and ethically to create a more sustainable and fair future. By embracing these advancements, we can drive positive change, build global partnerships, and create a world where AI supports humanity’s biggest dreams and goals.
In your opinion, what are the most promising use cases for generative AI in the banking and telecommunications sectors?
Banking:
Overall, generative AI can transform banking by creating a more engaging customer experience through personalized communication and by streamlining operations with automated tasks and risk management.
· Client Engagement and Communication: The report highlights the potential for generative AI to redefine a bank’s competitive edge in client relationships. Large language models can power chatbots and virtual assistants that provide personalized and efficient communication, fostering trust and loyalty with customers. Imagine chatbots that can answer complex financial questions, schedule appointments, or even negotiate loan terms in a natural and engaging way.
· Risk Management and Compliance: This area is a top priority for banks according to the report. Generative AI can analyze vast amounts of data to identify and mitigate financial risks, detect fraud, and ensure adherence to regulations. For example, AI can analyze transaction patterns to identify suspicious activity or generate reports that comply with complex financial regulations.
· Workforce Transformation: Generative AI can automate repetitive tasks currently handled by bank employees, freeing them to focus on more complex areas or client interactions. This can streamline operations, improve efficiency, and potentially allow employees to provide higher-quality service. Tasks like generating routine reports or processing loan applications could be automated by generative AI.
More information can be found here
Telecommunication:
Overall, generative AI has the potential to revolutionize customer service in the telecommunications industry by providing a more personalized, efficient, and effective experience. The key use cases in this industry are:
· Customer Service: Generative AI can be used to create chatbots and virtual agents that can answer customer questions more effectively, understand complex inquiries, and provide a more natural conversational experience.
· Personalized Offers and Recommendations: Generative AI can analyze customer data to recommend new products, services, or plans that are tailored to their individual needs.
· Network Optimization: Generative AI can be used to analyze network data and identify potential problems before they occur, helping to improve network performance and reliability.
· Content Creation: Generative AI can be used to create personalized videos or other content that explains invoices, service changes, or other complex topics to customers in a clear and easy-to-understand way.
· Call Summaries and Analysis: Generative AI can be used to generate summaries of customer calls, which can then be used to identify trends and improve customer service processes.
More information can be found here
AI governance is a critical aspect of your expertise. How do you ensure ethical and responsible AI practices in the projects you lead?
Absolutely! watsonx.governance empowers me to ensure ethical and responsible AI practices in the projects I lead. Here’s how:
· Transparency and Explainability: I leverage watsonx.governance’s AI use cases to track assets throughout their lifecycle. Factsheets within these use cases capture details about the AI model or prompt template, including its development process and training data. This fosters transparency and allows human oversight at every stage.
· Fairness and Non-discrimination: watsonx.governance helps me mitigate bias in AI assets. It allows for monitoring of deployed models for fairness drift, ensuring the model’s outputs remain unbiased over time. Additionally, I can use the AI Risk Atlas, a resource within watsonx.governance, to identify potential fairness risks early in the development process.
· Safety and Security: watsonx.governance provides tools to monitor generative AI assets for breaches of toxic language thresholds or detection of personal identifiable information (PII). This helps to safeguard against the generation of harmful content and protects user privacy.
· Accountability and Human Oversight: Human oversight remains crucial. watsonx.governance facilitates the creation of AI use cases, which assign roles and responsibilities throughout the AI lifecycle. This ensures clear ownership and accountability for AI decisions.
· Privacy and Data Protection: watsonx.governance integrates with IBM OpenPages Model Risk Governance, which allows for the collection of metadata about foundation models. This comprehensive view of AI assets empowers informed decisions regarding data privacy and helps ensure compliance with relevant regulations.
By employing these capabilities within watsonx.governance, I can ensure that the AI projects I lead are not only effective but also adhere to the highest ethical standards.”
Can you discuss a specific generative AI pilot that you’ve worked on? What were the key factors that contributed to its success?
Yes I worked on this project. I’m pretty limited in what I can share unfortunately. More information can be found here
What advice would you give to businesses selecting use cases for their initial generative AI pilots to maximize their chances of success?
Here’s my advice to maximize your chances of success with generative AI pilots:
Start with a structured approach for success.
- Build Expertise: Assemble a team comfortable with generative AI for experimentation.
- Test with Low-Risk Use Case: Choose an internal project for initial prototyping and deployment. Consider using a free trial of IBM watsonx to gain experience.
- Define Value Drivers: Discuss factors like trustworthiness, regulatory compliance, and value metrics. Choose metrics that reflect business goals and measure robustness, fairness, scalability, and deployment cost.
- Start Simple with Pre-Trained Models: Begin with pre-trained models and customize them with your data for faster implementation.
Beyond Cost Savings, Aim for Growth:
Once you’ve received organizational buy-in and a quick wins in your low risk use case, it’s time to focus on high-impact use cases that drive growth, not just cost savings:
· While cost reduction is attractive for quick wins, it alone won’t lead to significant growth.
· Look for use cases that can transform your business and provide a competitive advantage. We often look at GenAI solutions that “mimic” human work. However, Generative AI shouldn’t replace human roles; it should augment them.
Think of Generative AI as a much more impactful tool to:
· Expand the realm of human possibilities: Enhance knowledge and imagination by creating solutions to unsolved problems.
· Address future needs: Open opportunities by tackling challenges we haven’t yet realized.
· Use the ” Airbnb 11-star method” to identify breakthrough ideas:
- This method asks you to consider what aspects of your business customers and employees value most.
- Imagine improvements beyond a 5-star rating, all the way to an “11-star” experience.
- This can spark creative thinking for how generative AI can enhance those experiences.
Focusing solely on cost-saving GenAI applications risks missing the true value. By not exercising our imagination, we get caught in the hype and miss out on GenAI’s true potential: to go beyond replicating human work.
By following these steps and focusing on growth and human potential, businesses can increase their chances of success with generative AI pilots, achieving results that extend far beyond chatbots.
Looking ahead, what do you predict will be the next major advancements in AI and machine learning that will drive digital transformation in the next five years?
These are just a few of the many advancements in AI and machine learning that I’m expecting to see in the next five years. These advancements will have a profound impact on all aspects of our lives, from the way we work to the way we live.
1. Reinventing Products: Richer, More Intelligent, Embedded
A new paradigm of how we interact with our products. Imagine everyday products infused with AI, making them not just smarter but fundamentally reimagined. Here are some examples:
- Smart Fridge: Your refrigerator that learns your eating habits and preferences, automatically generating shopping lists based on what’s running low. It can even suggest recipes based on the ingredients you have on hand and display them on a built-in screen. Imagine an oven that preheats to the perfect temperature based on the recipe you’ve selected on the fridge’s screen.
- AI-Powered Oven: This oven doesn’t just preheat; it understands what’s being cooked. Using sensors and image recognition, it can automatically adjust settings for perfect results, and even alert you if something is at risk of burning.
- Self-Diagnosing Washing Machine: Imagine a washing machine that can identify potential problems, like a clogged drain or an unbalanced load. It can then offer tips on better detergent use for different types of clothes, and even schedule an appointment with a service agent through its connected app.
- AI-Enhanced Car: Take the concept of a built-in GPS a step further. Imagine a car with a conversational AI system like ChatGPT built-in. Peugeot recently launched this I believe. You can ask your car for 4-star rated restaurants nearby that specialize in fish dishes. `
This “embedded edge” intelligence will blur the lines between the physical and digital, creating a richer and more intuitive user experience.
2. Intelligent AI Agents
· Advanced AI Agents: AI agents are expected to become more intelligent and ubiquitous. Imagine AI agents with:
· Level 5 intelligence: This means cognitive abilities such as memory, complex reasoning, and autonomous learning.
· Interconnectivity: Seamlessly collaborating with each other and accessing vast amounts of data to offer the most comprehensive assistance possible.
This means they will be able to learn and adapt to new situations more quickly, and they will be embedded in a wider range of devices and applications.
3. AI Governance
As AI becomes more sophisticated, ethical considerations and responsible use become more important. Here’s what we can expect:
- Invisible AI watermarks: Tracking the origin and purpose of AI interactions for transparency and accountability.
- Safewords: Mechanisms for users to easily opt-out of AI interactions or request human intervention.
- More legislation: Regulatory frameworks like the AI EU Act will establish clear guidelines for responsible AI development and deployment
4. Quantum Computing: The Long Game
While not an immediate game-changer, quantum computing holds immense potential in the long run. Quantum computers can tackle problems with multiple complex factors, something that overwhelms traditional computers.
This opens doors to breakthroughs in areas like optimizing calculations: Imagine designing materials with previously unheard-of properties, drug discovery, or developing new financial models that account for a multitude of variables with unmatched accuracy. Quantum computing represents a paradigm shift in computing power, paving the way for advancements that could fundamentally change the world around us.