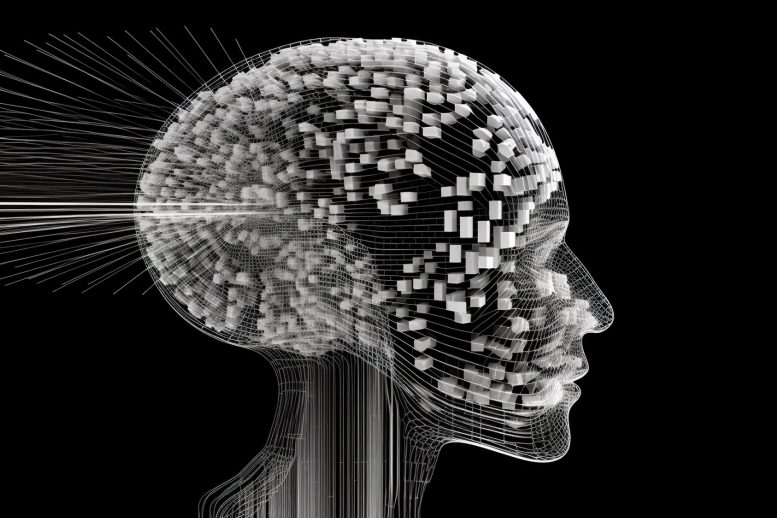
A study from Bar-Ilan University reveals that the brain’s efficient shallow learning, involving a wide network with few layers, can compete with the multi-layered deep learning models in complex classification tasks. This challenges the current design of GPUs, which favor deep over wide architectures.
The brain, despite its comparatively shallow structure with limited layers, operates efficiently, whereas modern AI systems are characterized by deep architectures with numerous layers. This raises the question: Can brain-inspired shallow architectures rival the performance of deep architectures, and if so, what are the fundamental mechanisms that enable this?
Neural network learning methods are inspired by the brain’s functioning, yet there are fundamental differences between how the brain learns and how deep learning operates. A key distinction lies in the number of layers each employs.
Deep learning systems often have many layers, sometimes extending into the hundreds, which allows them to effectively learn complex classification tasks. In contrast, the human brain has a much simpler structure with far fewer layers. Despite its relatively shallow architecture and the slower, noisier nature of its processes, the brain is remarkably adept at handling complex classification tasks efficiently.
Research on Shallow Learning Mechanisms in the Brain
The key question driving new research is the possible mechanism underlying the brain’s efficient shallow learning — one that enables it to perform classification tasks with the same accuracy as deep learning. In an article published in Physica A, researchers from Bar-Ilan University in Israel show how such shallow learning mechanisms can compete with deep learning.
Credit: Prof. Ido Kanter, Bar-Ilan University
“Instead of a deep architecture, like a skyscraper, the brain consists of a wide shallow architecture, more like a very wide building with only very few floors,” said Prof. Ido Kanter, of Bar-Ilan’s Department of Physics and Gonda (Goldschmied) Multidisciplinary Brain Research Center, who led the research.
“The capability to correctly classify objects increases where the architecture becomes deeper, with more layers. In contrast, the brain’s shallow mechanism indicates that a wider network better classifies objects,” said Ronit Gross, an undergraduate student and one of the key contributors to this work.
“Wider and higher architectures represent two complementary mechanisms,” she added. Nevertheless, the realization of very wide shallow architectures, imitating the brain’s dynamics, requires a shift in the properties of advanced GPU technology, which is capable of accelerating deep architecture, but fails in the implementation of wide shallow ones.
Reference: “Efficient shallow learning mechanism as an alternative to deep learning” by Ofek Tevet, Ronit D. Gross, Shiri Hodassman, Tal Rogachevsky, Yarden Tzach, Yuval Meir and Ido Kanter, 11 January 2024, Physica A: Statistical Mechanics and its Applications.
DOI: 10.1016/j.physa.2024.129513