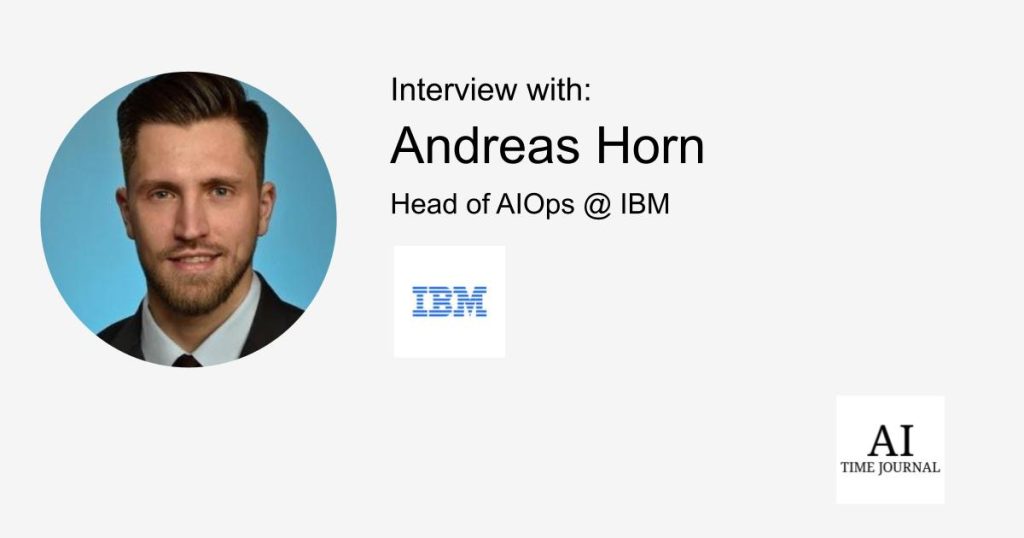
In this compelling conversation, Andreas Horn, Head of AIOps at IBM, delves into the transformative role of AI in modern business operations. With IBM leading the charge in AI and automation, Andreas shares his perspectives on the challenges of AI adoption, from ensuring secure and scalable systems to integrating AI within legacy infrastructures. He also discusses the future of work in an AI-driven world, the ethical considerations businesses must navigate, and IBM’s strategic use of Generative AI in AIOps. Explore Andreas’ vision for the next frontier in AIOps and what it means for the future of digital transformation.
As Head of AIOps at IBM, how do you see the evolving role of AI and automation in transforming traditional business operations, and what challenges do organizations face in adopting these technologies at scale?
To answer this question, let’s look at the latest numbers. At IBM, we conducted more than 1,000 GenAI pilots over the past 12 months, with around 10-20% of those moving into production. We’re seeing a significant increase in AI projects, and use cases like retrieval-augmented generation (RAG) for knowledge management are demonstrating substantial value for many clients and scenarios. However, the key concern is always ROI. To succeed, AI must deliver real value by addressing customer pain points, making the business case essential.
For the second part of the question:
The main bottleneck is the lack of high-quality, accessible data and the complexity of managing data effectively. High-quality data is essential, but often it’s missing or inadequate. The phrase “garbage in, garbage out” is especially true when it comes to AI implementation. I often see companies focusing on building their AI strategy, but in my view, you need a clear data strategy in place before developing an AI strategy.
There are also other key challenges, such as a significant skills gap, as there is a shortage of AI expertise (especially in the European market). Additionally, integrating AI with legacy systems (change management), addressing ethical concerns, and managing the high costs of implementation are major hurdles.
With your expertise in AIOps, how do you ensure that AI systems remain robust, scalable, and secure as they are integrated into complex business environments?
I believe three key factors are crucial for success. First and foremost, securing the business environment is essential, especially when handling sensitive data. This means protecting user access, defending against outside security threats, and implementing real-time performance tracking with automated alerts. These measures help quickly identify and address any potential security issues.
It’s also vital to establish a strong architecture with robust data governance practices. I said it before: Having your data in place is unfortunately often overlooked and a bottleneck. Using data management tools to ensure data integrity and accessibility is crucial. Seamless integration is key, as AI systems must work in harmony with existing processes and technology. Equally important is AI governance, where clear policies are set to manage compliance with legal, ethical, and data standards, as well as model management.
Finally, for deployment and monitoring, I advocate for an open, trusted hybrid cloud infrastructure. This architecture allows AI models to be utilized across the organization, enabling secure collaboration between various business units. We also implement automated scaling to adjust resources based on demand, ensuring optimal performance even as workloads fluctuate.
AI, automation, and security intersection is critical in today’s digital landscape. How do you approach the integration of DevSecOps principles within AIOps to maintain security without hindering innovation?
We approach the integration of DevSecOps principles within AIOps by adopting a “shift-left” security strategy. This means incorporating automated security testing early in the development process, treating security as code, and catching vulnerabilities before they become major issues. AI-powered security analytics play a big role in enhancing threat detection and enabling predictive security measures, while continuous compliance monitoring automates governance and keeps processes in check.
Equally important is fostering a collaborative security culture. We involve security experts in cross-functional teams and provide ongoing training to ensure security is everyone’s responsibility.
How do you foresee the future of work evolving with the rise of AI and automation, particularly regarding skillsets that will be in demand, and what advice would you give to professionals aiming to stay relevant in this new landscape?
First, it’s essential to realistically assess your current skillset, especially your understanding of AI and related technologies. Are you familiar with concepts like machine learning, deep learning, neural networks, and the differences between supervised, unsupervised, and reinforcement learning? Reflecting on your current knowledge will help you identify gaps and create a personalized learning plan. You can also ask more senior colleagues to support you in setting up a plan.
Starting with the basics is key, and there are plenty of free resources available to get you up to speed. For instance, IBM SkillBuild (free) offers a comprehensive platform for learning AI, and there are other valuable resources like LinkedIn, Amazon AI, Udemy, Coursera, and YouTube, where you can access tutorials and courses at no cost. I truly believe that the best material to upskill is available for free.
Beyond technical skills, soft skills will become increasingly important as AI automates more routine tasks. Critical thinking, creativity, and emotional intelligence will be crucial in areas where human judgment is still necessary. Additionally, as AI implementation often involves significant change management, professionals with strong people skills will be invaluable in guiding teams through these transitions.
My advice: stay curious, continuously learn, and focus on building a combination of technical and soft skills to remain relevant in this fast-changing landscape.
Generative AI has been a game-changer in many industries. How is IBM leveraging GenAI within its AIOps strategy, and what potential do you see for GenAI in optimizing business operations?
We’re using GenAI to enhance our predictive analytics capabilities. By training large language models on vast amounts of IT operations data, we can generate highly accurate forecasts of potential issues and automate root cause analysis. This proactive approach helps us address problems before they impact business operations, leading to greater efficiency and uptime. At IBM we have built several market-leading assets which are performing very well!
We are also improving our automated incident response systems. These models can quickly generate and suggest remediation steps based on historical data and current system states, significantly reducing the mean time to resolution and helping teams resolve issues faster.
In addition, we are optimizing resource allocation and cloud spending. Our AI models analyze usage patterns and provide tailored recommendations for distributing resources across hybrid cloud environments (FinOps), resulting in substantial cost savings for our clients.
Leadership in the AI and tech industry requires a unique blend of skills. How do you foster a culture of innovation and continuous learning among your team while leading AIOps initiatives at IBM?
I focus on building a culture rooted in a growth mindset. I encourage my team to view challenges as opportunities for growth and development. To foster innovation and continuous learning, I ensure my team has the freedom and time to focus on upskilling and expanding their knowledge. It’s equally important to give people the opportunity to experiment with new technologies, allowing them to explore ideas without the fear of failure.
Another very important aspect is to create forums for the exchange of these new discoveries and innovations for colleagues. At IBM, our people constantly find new tweaks and workflows to improve processes, especially with AI. Sharing those insights so others can benefit is crucial. To support this, we regularly hold technical deep dives, we organize rallies, workshops, and hackathons that bring together experts from various disciplines to spark innovative discussions.
Recognizing and crediting people for their outstanding work is also key. It not only boosts morale but reinforces the value of their contributions, helping to further fuel a culture of continuous improvement and creativity.
AI-driven automation is rapidly advancing. In your view, what are the most critical ethical considerations that businesses must address when implementing AIOps solutions, and how does IBM navigate these challenges?
At IBM, we strongly believe that AI should enhance human capabilities, not replace them. Many critical aspects need to be considered, such as data privacy and security. It’s also critical to tackle algorithmic bias by using diverse datasets and performing rigorous testing to ensure fair and unbiased outcomes.
Also important to consider is transparency and explainability in AI-driven decisions are essential for building trust with users and clients. We prioritize maintaining human oversight and control in automated systems to prevent unintended consequences. Additionally, we believe that all companies estimate the impact of automation on their workforce and invest in reskilling initiatives to prepare employees for new roles.
From a technical perspective at IBM, we are also developing solutions like WatsonX.governance to comprehensively address these challenges. Ethical and responsible AI is central to everything we do, ensuring that our AI initiatives are grounded in fairness, transparency, and accountability.
Integrating AI and automation often requires overcoming significant organizational resistance. How do you manage change and drive the adoption of AIOps technologies within IBM and with your clients?
I believe that technology accounts for only about 30% of success in IT projects, while 70% comes down to focusing on people and managing change effectively. To drive AIOps adoption, we prioritize education and awareness through regular workshops and training sessions, demonstrating real-world benefits in action. Collaboration is key, so we involve key stakeholders early in the process to ensure their concerns are addressed and their input is valued.
We often start with pilot projects to allow teams to gain confidence in the technology before scaling up. Throughout the transition, we provide strong support, including dedicated change management teams and clear communication channels to guide everyone through the process. Continuously measuring and communicating the impact of AIOps adoption helps reinforce its value and keep momentum going.
By focusing on the human element and managing change thoughtfully, we’ve found that organizations are much more successful in integrating AIOps.
What role do you believe AIOps will play in shaping the future of digital transformation, and how is IBM positioning itself to lead in this rapidly changing landscape?
I see AIOps as a critical driver of digital transformation, especially as IT departments typically allocate around 70% of their budgets to operations. This presents a huge opportunity for optimization and efficiency. As businesses become increasingly digital, the complexity of IT operations grows exponentially, and we need solutions that can simplify and optimize these systems.
At IBM, we recognize the importance of AIOps and have made significant investments to lead in this space. With over $10 billion invested in acquiring tools like Apptio, Instana, Turbonomic, and SevOne, along with the development of our own AIOps platforms, our goal is to maintain momentum and expand our leading role in the field.
As someone deeply involved in the strategic application of AI and automation, what do you see as the next big frontier in AIOps, and how should organizations prepare for these upcoming advancements?
I see the next big frontier in AIOps as the rise of AI agents and multi-agent systems capable of autonomously solving problems. Our long-term vision is to develop autonomous IT operations systems, achieving zero-touch operations and self-healing capabilities. This is our moonshot — it may take 8-10 years to fully realize, but the exponential growth of AI could accelerate this timeline.
To prepare for these advancements, organizations should prioritize building a solid data foundation and developing their AI capabilities. Investing in upskilling the workforce to collaborate effectively with advanced AI systems will be key. Additionally, fostering a culture of innovation and continuous learning will help organizations adapt to the rapidly evolving AIOps landscape.