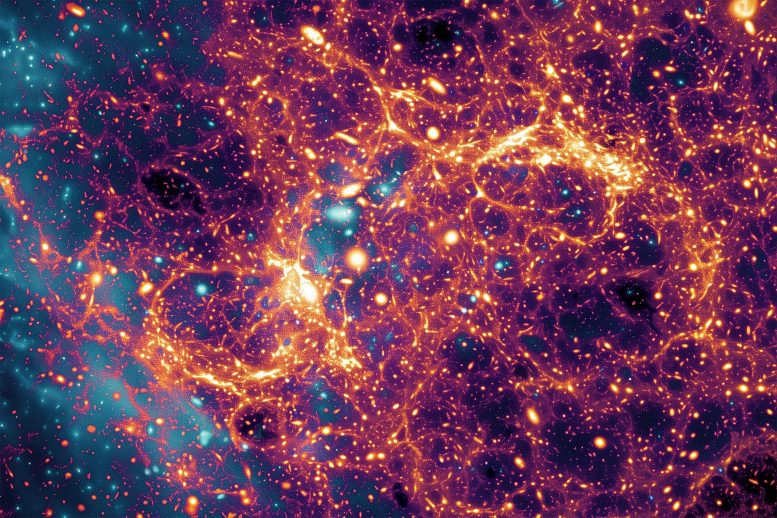
The Dark Energy Survey has enhanced our understanding of the Universe, doubling the precision of dark energy measurements through AI and simulation techniques, and offering insights into the Universe’s structure and the potential need for new cosmological models. Credit: SciTechDaily.com
A UCL-led research team has used artificial intelligence (AI) techniques to infer the influence and properties of dark energy more precisely from a map of dark and visible matter in the Universe covering the last seven billion years.
The study, carried out by the Dark Energy Survey collaboration, doubled the precision at which key characteristics of the Universe, including the overall density of dark energy, could be inferred from the map.
This increased precision allows researchers to rule out models of the Universe that might previously have been conceivable.
Advances in Cosmic Understanding
Dark energy is the mysterious force that is accelerating the Universe’s expansion and is thought to make up about 70% of the content of the Universe (with dark matter, invisible stuff whose gravity pulls galaxies, making up 25%, and normal matter just 5%).
Lead author Dr. Niall Jeffrey (UCL Physics & Astronomy) said: “Using AI to learn from computer-simulated universes, we increased the precision of our estimates of key properties of the Universe by a factor of two.
“To achieve this improvement without these novel techniques, we would need four times the amount of data. This would be equivalent to mapping another 300 million galaxies.”
Co-author Dr. Lorne Whiteway (UCL Physics & Astronomy) said: “Our findings are in line with the current best prediction of dark energy as a ‘cosmological constant’ whose value does not vary in space or time. However, they also allow flexibility for a different explanation to be correct. For instance, it still could be that our theory of gravity is wrong.”
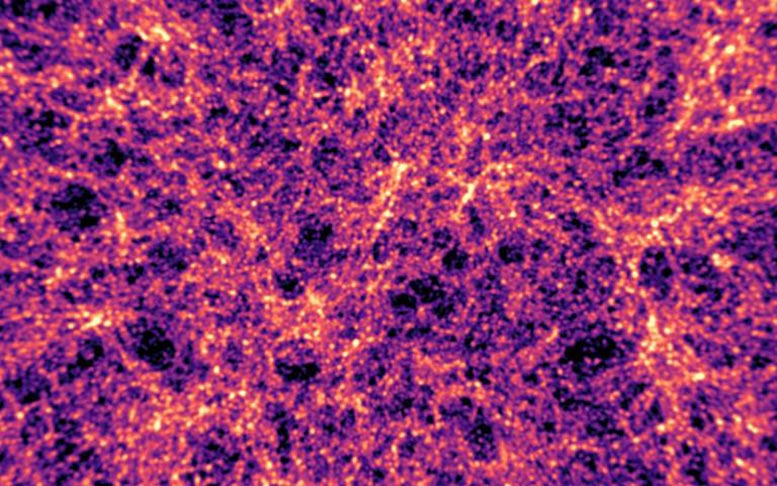
A matter map derived from one of the simulated universes. The lightest areas of the map show the regions where dark matter is most dense. These correspond to superclusters of galaxies. The dark, almost black patches are cosmic voids, the large empty spaces in between clusters of galaxies. Credit: Niall Jeffrey et al
Refining Cosmological Models
In line with previous analysis of the Dark Energy Survey map, first published in 2021, the findings suggest that matter in the Universe is more smoothly spread out – less lumpy – than Einstein’s theory of general relativity would predict. However, the discrepancy was less significant for this study compared to the earlier analysis, as the error bars were larger.
The Dark Energy Survey map was obtained through a method called weak gravitational lensing – that is, seeing how light from distant galaxies has been bent by the gravity of intervening matter on its way to Earth.
The collaboration analyzed distortions in the shapes of 100 million galaxies to infer the distribution of all matter, both dark and visible, in the foreground of those galaxies. The resulting map covered a quarter of the sky in the Southern Hemisphere.
For the new study, researchers used UK government-funded supercomputers to run simulations of different universes based on the data from the Dark Energy Survey matter map. Each simulation had a different mathematical model of the universe underpinning it.
The researchers created matter maps from each of these simulations. A machine learning model was used to extract the information in those maps that was relevant to cosmological models. A second machine learning tool, learning from the many examples of simulated universes with different cosmological models, looked at the real observed data and gave the odds on any cosmological model being the true model of our Universe.
This new technique allowed researchers to use much more information from the maps than would be possible with the previous method.
The simulations were run on DiRAC High Performance Computing (HPC) facility, funded by the UK’s Science and Technology Facilities Council (STFC).
Future Explorations in Cosmology
The next phase of dark universe projects – including the European Space Agency (ESA) mission Euclid, launched last summer – will greatly increase the quantity of data we have on the large-scale structures of the Universe, helping researchers determine if the unexpected smoothness of the Universe is a sign current cosmological models are wrong or if there is another explanation for it.
Currently, this smoothness is at odds with what would be predicted based on analysis of the cosmic microwave background (CMB) – the light left over from the Big Bang.
The Dark Energy Survey collaboration, of which UCL is a founding member, is hosted by the US Department of Energy’s Fermi National Accelerator Laboratory (Fermilab) and involves more than 400 scientists from 25 institutions in seven nations.
The collaboration has cataloged hundreds of millions of galaxies, using photographs of the night sky taken by the 570-megapixel Dark Energy Camera, one of the world’s most powerful digital cameras, over six years (from 2013 to 2019). The camera, whose optical corrector was built at UCL, is mounted on a telescope at the National Science Foundation’s Cerro Tololo Inter-American Observatory in Chile.
Reference: “Dark Energy Survey Year 3 results: likelihood-free, simulation-based wCDM inference with neural compression of weak-lensing map statistics” by N. Jeffrey, L. Whiteway, M. Gatti, J. Williamson, J. Alsing, A. Porredon, J. Prat, C. Doux, B. Jain, C. Chang, T.-Y. Cheng, T. Kacprzak, P. Lemos, A. Alarcon, A. Amon, K. Bechtol, M. R. Becker, G. M. Bernstein, A. Campos, A. Carnero Rosell, R. Chen, A. Choi, J. DeRose, A. Drlica-Wagner, K. Eckert, S. Everett, A. Ferté, D. Gruen, R. A. Gruendl, K. Herner, M. Jarvis, J. McCullough, J. Myles, A. Navarro-Alsina, S. Pandey, M. Raveri, R. P. Rollins, E. S. Rykoff, C. Sánchez, L. F. Secco, I. Sevilla-Noarbe, E. Sheldon, T. Shin, M. A. Troxel, I. Tutusaus, T. N. Varga, B. Yanny, B. Yin, J. Zuntz, M. Aguena, S. S. Allam, O. Alves, D. Bacon, S. Bocquet, D. Brooks, L. N. da Costa, T. M. Davis, J. De Vicente, S. Desai, H. T. Diehl, I. Ferrero, J. Frieman, J. García-Bellido, E. Gaztanaga, G. Giannini, G. Gutierrez, S. R. Hinton, D. L. Hollowood, K. Honscheid, D. Huterer, D. J. James, O. Lahav, S. Lee, J. L. Marshall, J. Mena-Fernández, R. Miquel, A. Pieres, A. A. Plazas Malagón, A. Roodman, M. Sako, E. Sanchez, D. Sanchez Cid, M. Smith, E. Suchyta, M. E. C. Swanson, G. Tarle, D. L. Tucker, N. Weaverdyck, J. Weller, P. Wiseman and M. Yamamoto, 4 March 2024, Cosmology and Nongalactic Astrophysics.
arXiv:2403.02314