Dario Wünsch was feeling assured. The 28-year-old from Leipzig, Germany, was about to change into the primary skilled gamer to tackle the factitious intelligence program AlphaStar within the rapid-fire online game StarCraft II. Wünsch had been professionally enjoying StarCraft II, wherein opponents command alien fleets vying for territory, for almost a decade. No means may he lose this five-match problem to a newly minted AI gamer.
Even AlphaStar’s creators on the London-based AI analysis firm DeepMind, which is a part of Alphabet, Inc., weren’t optimistic in regards to the end result. They have been the most recent in a protracted line of researchers who had tried to construct an AI that might deal with StarCraft II’s dizzying complexity. To this point, nobody had created a system that might beat seasoned human gamers.
Certain sufficient, when AlphaStar confronted off in opposition to Wünsch on December 12, the AI appeared to commit a deadly mistake on the onset of the primary match: It uncared for to construct a protecting barrier on the entrance to its camp, permitting Wünsch to infiltrate and shortly choose off a number of of its employee models. For a minute, it appeared like StarCraft II would stay one realm the place people trump machines. However AlphaStar made a profitable comeback, assembling a tenacious strike crew that shortly laid waste to Wünsch’s defenses. AlphaStar 1, Wünsch 0.
Wünsch shook it off. He simply wanted to focus extra on protection. However within the second spherical, AlphaStar shocked the professional gamer by withholding assaults till it had amassed a military that after once more crushed Wünsch’s forces. Three matches later, AlphaStar had received the competitors 5-0, relegating Wünsch to the small however rising membership of world-class avid gamers bested by a machine.
Researchers have lengthy used video games as benchmarks for AI smarts. In 1997, IBM’s Deep Blue earned worldwide acclaim by outwitting chess champion Garry Kasparov (SN: 8/2/97, p. 76). In 2016, DeepMind’s AlphaGo famously overpowered Go champion Lee Sedol (SN: 12/24/16, p. 28).
However board-based contests like chess and Go can solely push AI up to now. These video games are nonetheless fairly easy — gamers take turns and may see each piece’s place on the board always. In relation to making an AI that may cope with real-world ambiguity and fast-paced interactions, essentially the most helpful exams of machine cognition will most likely be present in video games performed in digital worlds.
Constructing AI avid gamers that may trounce human gamers is greater than a conceit challenge. “The final word thought is to … use these algorithms [for] real-world challenges,” says Sebastian Risi, an AI researcher at IT College of Copenhagen. As an illustration, after the San Francisco–based mostly firm OpenAI educated a five-AI squad to play a web-based battle recreation referred to as Dota 2, the programmers repurposed these algorithms to show the 5 fingers of a robotic hand to manipulate objects with unprecedented dexterity. The researchers described this work on-line at arXiv.org in January.
DeepMind researchers equally hope that AlphaStar’s design may inform researchers making an attempt to construct AIs to deal with lengthy sequences of interactions, like these concerned in simulating local weather change or understanding conversation, an particularly tough process (SN: 3/2/19, p. 8).
Proper now, two vital issues that AIs nonetheless wrestle with are: coordinating with one another and frequently making use of new information to new conditions. The StarCraft universe has proved to be a wonderful testing floor for strategies that make AI extra cooperative. To experiment with strategies to make AIs ceaselessly learners, researchers are utilizing one other widespread online game, Minecraft. Whereas folks might use display time as an entertaining distraction from actual life, digital challenges might assist AI choose up the abilities mandatory to reach the actual world.
Crew play
When AlphaStar took on Wünsch, the AI performed StarCraft II like a human would: It acted like a single puppeteer with full management over all of the characters in its fleet. However there are various real-world conditions wherein counting on one mastermind AI to micromanage plenty of units would change into unwieldy, says synthetic intelligence researcher Jakob Foerster of Fb AI Analysis in San Francisco.
Consider overseeing dozens of nursing robots caring for sufferers all through a hospital, or self-driving vehicles coordinating their speeds throughout miles of freeway to mitigate site visitors bottlenecks. So, researchers together with Foerster are utilizing the StarCraft video games to check out totally different “multiagent” schemes.
In some designs, particular person fight models have some independence, however are nonetheless beholden to a centralized controller. On this setup, the overseer AI acts like a coach shouting performs from the sidelines. The coach generates a big-picture plan and points directions to crew members. Particular person models use that steerage, together with detailed observations of the rapid environment, to determine act. Laptop scientist Yizhou Wang of Peking College in China and colleagues reported the effectiveness of this design in a paper submitted to IEEE Transactions on Neural Networks and Studying Programs.
Wang’s group educated its AI crew in StarCraft utilizing reinforcement studying, a kind of machine studying wherein laptop methods choose up abilities by interacting with the setting and getting digital rewards after doing one thing proper. Every teammate acquired rewards based mostly on the variety of enemies eradicated in its rapid neighborhood and whether or not your entire crew received in opposition to fleets managed by an automatic opponent constructed into the sport. On a number of totally different challenges with groups of at the least 10 fight models, the coach-guided AI groups received 60 to 82 p.c of the time. Centrally managed AI groups with no capability for unbiased reasoning have been much less profitable in opposition to the built-in opponent.
AI crews with a single commander in chief that exerts at the least some management over particular person models may fit greatest when the group can depend on quick, correct communication amongst all brokers. As an illustration, this technique may work for robots inside the similar warehouse.
However for a lot of machines, similar to self-driving automobiles or drone swarms unfold throughout huge distances, separate units “received’t have constant, dependable and quick knowledge connection to a single controller,” Foerster says. It’s each AI for itself. AIs working beneath these constraints usually can’t coordinate in addition to centralized groups, however Foerster and colleagues devised a coaching scheme to organize independent-minded machines to work collectively.
On this system, a centralized observer affords suggestions to teammates throughout reinforcement studying. However as soon as the group is absolutely educated, the AIs are on their very own. The grasp agent is much less like a sidelined coach and extra like a dance teacher who affords ballerinas pointers throughout rehearsals, however stays mum through the onstage efficiency.
The AI overseer prepares particular person AIs to be self-sufficient by providing customized recommendation throughout coaching. After every trial run, the overseer simulates different potential futures and tells every agent, “That is what truly occurred, and that is what would have occurred if everybody else had executed the identical factor, however you probably did one thing totally different.” This technique, which Foerster’s crew introduced in New Orleans in February 2018 on the AAAI Convention on Synthetic Intelligence, helps every AI unit decide which actions assist or hinder the group’s success.
To check this framework, Foerster and colleagues educated three teams of 5 AI models in StarCraft. Skilled models needed to act based mostly solely on observations of the rapid environment. In fight rounds in opposition to similar groups commanded by a built-in, nonhuman opponent, all three AI teams received most of their rounds, performing about in addition to three centrally managed AI groups in the identical fight situations.
Lifelong studying
The sorts of AI coaching that programmers check in StarCraft and StarCraft II are geared toward serving to a crew of AIs grasp a single process, for instance, coordinating site visitors lights or drones. The StarCraft video games are nice for that, as a result of for all their shifting components, the video games are pretty simple: Every participant has the singular purpose of overpowering an opponent. But when synthetic intelligence goes to change into extra versatile and humanlike, packages want to have the ability to be taught extra and frequently choose up new abilities.
“All of the methods that we see proper now that play Go and chess — they’re principally educated to do that one process effectively, after which they’re mounted to allow them to’t change,” Risi says. A Go-playing system introduced with an 18-by-18 grid, as a substitute of the usual 19-by-19 recreation board, would most likely must be utterly retrained on the brand new board, Risi says. Altering the traits of StarCraft models would require the identical back-to-square-one coaching. The Lego-like realm of Minecraft seems to be a greater place for testing approaches to make AI extra adaptable.
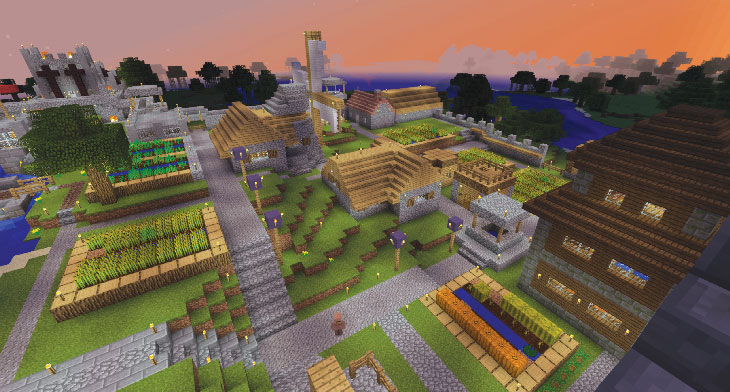
Not like StarCraft, Minecraft poses no single quest for gamers to finish. On this digital world fabricated from 3-D blocks of grime, glass and different supplies, gamers collect sources to construct buildings, journey, hunt for meals and do just about no matter else they please. Caiming Xiong, a synthetic intelligence researcher on the San Francisco–based mostly software program firm Salesforce, and colleagues used a easy constructing stuffed with blocks in Minecraft to check an AI designed to repeatedly be taught.
Moderately than assigning the AI to be taught a single process by means of trial and error in reinforcement studying, Xiong’s crew staggered the AI’s schooling. The researchers guided the AI by means of more and more tough reinforcement studying challenges, from discovering particular blocks to stacking blocks. The AI was designed to interrupt every problem into easier steps. It may sort out every step utilizing previous experience or attempt one thing new. In contrast with one other AI that was not designed to make use of prior information to tell new studying experiences, Xiong crew’s AI proved a a lot faster examine.
The knowledge-accumulating AI was additionally higher at adjusting to new conditions. Xiong and colleagues taught each AIs choose up blocks. Whereas coaching in a easy room that contained just one block, each AIs bought the “gather merchandise” ability down pat. However in a room with a number of blocks, the discrete-task AI struggled to establish its goal and grabbed the correct block solely 29 p.c of the time.
The knowledge-accumulating AI knew to rely on a previously learned “find item” skill to find a goal object amongst distractions. It picked up the correct block 94 p.c of the time. The analysis was introduced in Vancouver in Might 2018 on the Worldwide Convention on Studying Representations.
With additional coaching, Xiong and colleagues’ system may grasp extra abilities. However this design is restricted by the truth that the AI can solely be taught duties assigned by the human programmer throughout coaching. People don’t have this sort of instructional cutoff. When folks end faculty, “it’s not like, ‘Now you’re executed studying. You’ll be able to freeze your mind and go,’ ” Risi says.
A greater AI would get a foundational schooling in video games and simulations after which be capable to proceed studying all through its lifetime, says Priyam Parashar, a roboticist on the College of California, San Diego. A family robotic, for instance, ought to be capable to discover navigational work-arounds if residents set up child gates or rearrange the furnishings.
Parashar and colleagues created an AI that may establish cases wherein it wants additional coaching with out human enter. When the AI runs into a brand new impediment, it takes inventory of how the setting is totally different from what it anticipated. Then it will probably mentally rehearse varied work-arounds, think about the result of every and select one of the best answer.
The researchers examined this technique with an AI in a two-room Minecraft constructing. The AI had been educated to retrieve a gold block from the second room. However one other Minecraft participant had constructed a glass barrier within the doorway between the rooms, blocking the AI from accumulating the gold block. The AI assessed the scenario and, by means of reinforcement studying, figured out how to shatter the glass to complete its task, Parashar and her colleagues reported within the 2018 Data Engineering Evaluate.
An AI confronted with an sudden child gate or glass wall ought to most likely not conclude that one of the best answer is to bust it down, Parashar admits. However programmers can add extra constraints to an AI’s psychological simulations — just like the information that useful or owned objects shouldn’t be damaged — to tell the system’s studying, she says.
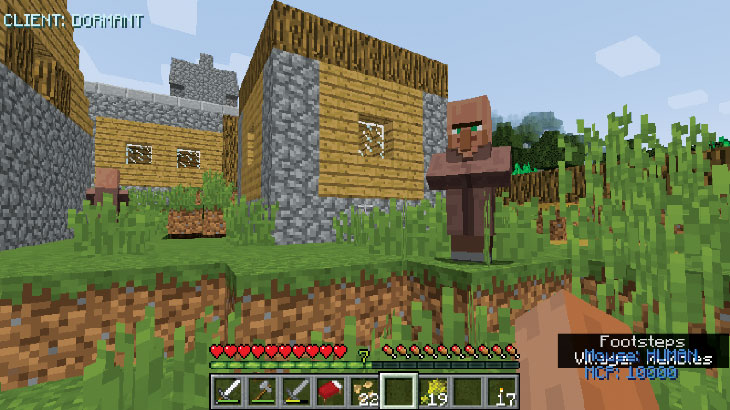
New video video games have gotten AI test-beds on a regular basis. AI and video games researcher Julian Togelius of New York College and colleagues hope to check collaborating AIs in Overcooked — a crew cooking recreation that takes place in a decent, crowded kitchen the place gamers are always getting in one another’s means. “Video games are designed to problem the human thoughts,” Togelius says. Any online game by nature is a ready-made check for the way a lot AI know-how can emulate human cleverness.
However with regards to testing AI in video video games or different simulated worlds, “you can not ever say, ‘OK, I’ve modeled all the pieces that’s going to occur in the actual world,’ ” Parashar says. Bridging the hole between digital and bodily actuality will take extra analysis.
One solution to hold simulation-trained AI from overreaching, she suggests, is to plot methods that require AIs to ask humans for help when needed (SN: 3/2/19, p. 8). “Which, in a way, is making [AI] extra like people, proper?” Parashar says. “We get by with the assistance of our pals.”