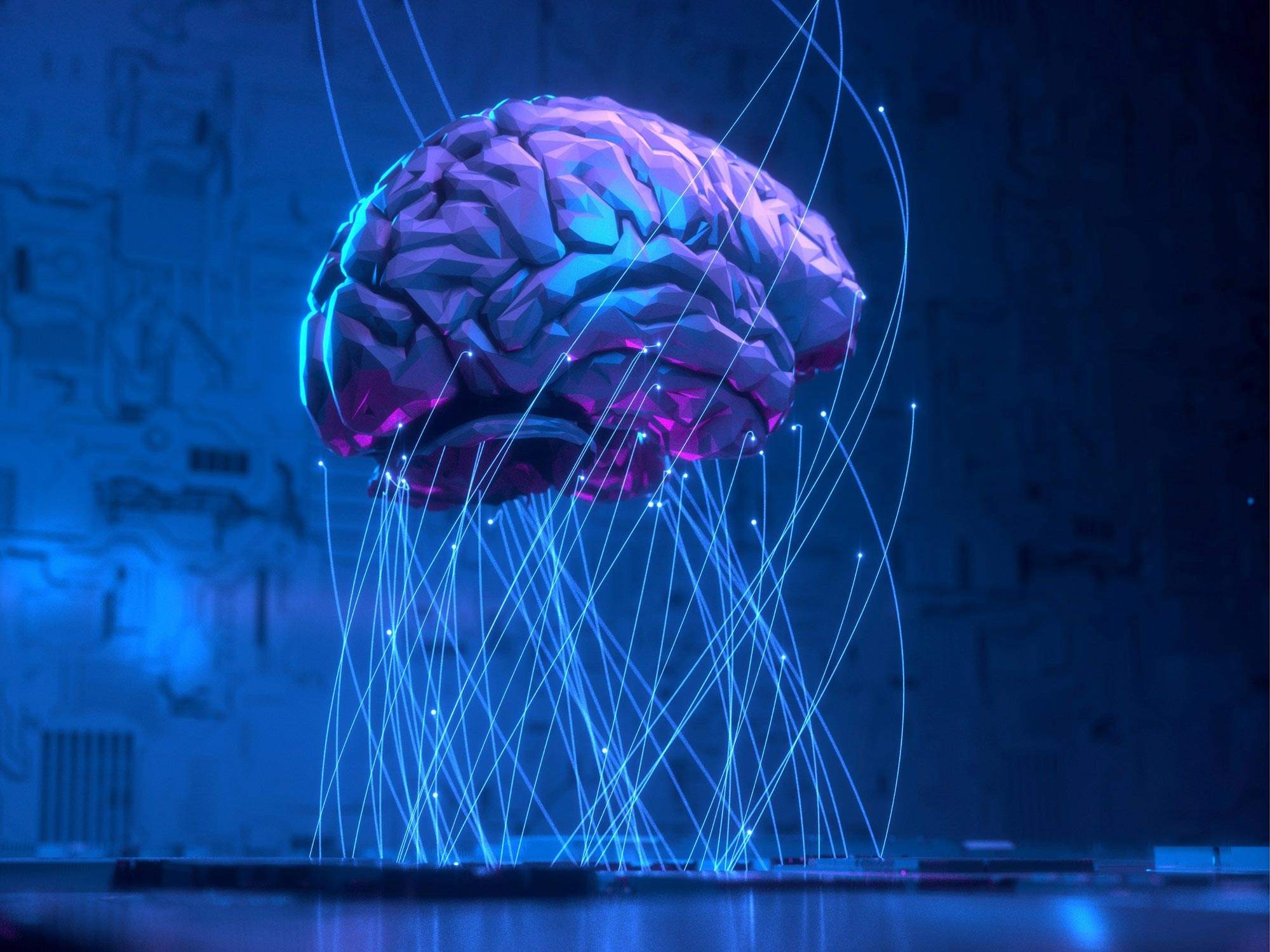
A analysis staff has demonstrated that overparametrization improves efficiency in quantum machine studying, a method that surpasses the capabilities of classical computer systems. Their analysis provides insights for optimizing the coaching course of in quantum neural networks, permitting for enhanced efficiency in sensible quantum purposes.
Extra is healthier — to some extent — when utilizing numerous parameters to coach machine-learning fashions on quantum computer systems.
A groundbreaking theoretical proof reveals that utilizing a method referred to as overparametrization enhances efficiency in quantum machine studying for duties that problem conventional computer systems.
“We imagine our outcomes might be helpful in utilizing machine studying to study the properties of quantum information, resembling classifying totally different phases of matter in quantum supplies analysis, which may be very tough on classical computer systems,” stated Diego Garcia-Martin, a postdoctoral researcher at Los Alamos National Laboratory. He’s a co-author of a brand new paper by a Los Alamos staff on the method in Nature Computational Science.
Garcia-Martin labored on the analysis within the Laboratory’s Quantum Computing Summer time Faculty in 2021 as a graduate scholar from the Autonomous College of Madrid.
Machine studying, or synthetic intelligence, often entails coaching neural networks to course of data — information — and learn to clear up a given job. In a nutshell, one can consider the neural community as a field with knobs, or parameters, that takes information as enter and produces an output that is determined by the configuration of the knobs.
“Through the coaching section, the algorithm updates these parameters because it learns, looking for their optimum setting,” Garcia-Martin stated. “As soon as the optimum parameters are decided, the neural community ought to be capable to extrapolate what it discovered from the coaching situations to new and beforehand unseen information factors.”
Each classical and quantum AI share a problem when coaching the parameters, because the algorithm can attain a sub-optimal configuration in its coaching and stall out.
A leap in efficiency
Overparametrization, a widely known idea in classical machine studying that provides increasingly parameters, can forestall that stall-out.
The implications of overparametrization in quantum machine studying fashions have been poorly understood till now. Within the new paper, the Los Alamos staff establishes a theoretical framework for predicting the crucial variety of parameters at which a quantum machine studying mannequin turns into overparametrized. At a sure crucial level, including parameters prompts a leap in community efficiency and the mannequin turns into considerably simpler to coach.
“By establishing the idea that underpins overparametrization in quantum neural networks, our analysis paves the best way for optimizing the coaching course of and attaining enhanced efficiency in sensible quantum purposes,” defined Martin Larocca, the lead creator of the manuscript and postdoctoral researcher at Los Alamos.
By benefiting from elements of quantum mechanics resembling entanglement and superposition, quantum machine studying provides the promise of a lot higher pace, or quantum benefit, than machine studying on classical computer systems.
Avoiding traps in a machine studying panorama
For instance the Los Alamos staff’s findings, Marco Cerezo, the senior scientist on the paper and a quantum theorist on the Lab, described a thought experiment during which a hiker in search of the tallest mountain in a darkish panorama represents the coaching course of. The hiker can step solely in sure instructions and assesses their progress by measuring altitude utilizing a restricted GPS system.
On this analogy, the variety of parameters within the mannequin corresponds to the instructions accessible for the hiker to maneuver, Cerezo stated. “One parameter permits motion forwards and backwards, two parameters allow lateral motion, and so forth,” he stated. An information panorama would possible have greater than three dimensions, not like our hypothetical hiker’s world.
With too few parameters, the walker can’t completely discover and would possibly mistake a small hill for the tallest mountain or get caught in a flat area the place any step appears futile. Nevertheless, because the variety of parameters will increase, the walker can transfer in additional instructions in greater dimensions. What initially appeared as an area hill would possibly become an elevated valley between peaks. With the extra parameters, the hiker avoids getting trapped and finds the true peak or the answer to the issue.
Reference: “Concept of overparametrization in quantum neural networks” by Martín Larocca, Nathan Ju, Diego García-Martín, Patrick J. Coles and Marco Cerezo, 26 June 2023, Nature Computational Science.
DOI: 10.1038/s43588-023-00467-6
The examine was funded by LDRD at Los Alamos Nationwide Laboratory.