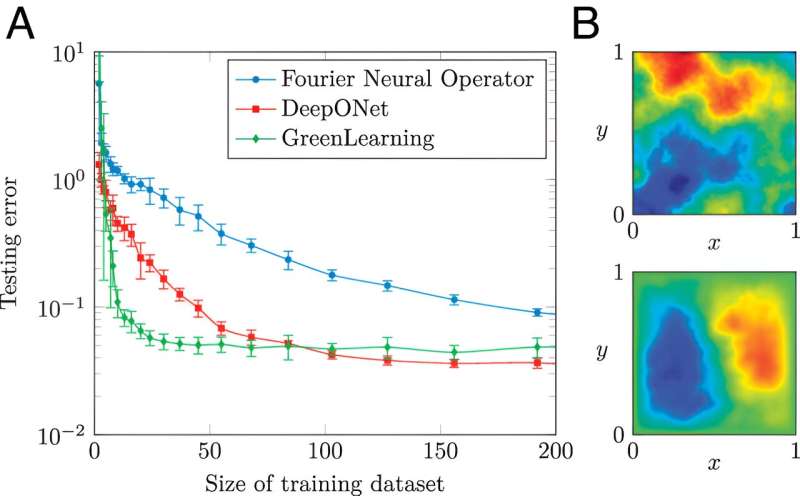
Researchers have decided find out how to construct dependable machine studying fashions that may perceive complicated equations in real-world conditions whereas utilizing far much less coaching information than is often anticipated.
The researchers, from the College of Cambridge and Cornell College, discovered that for partial differential equations—a category of physics equations that describe how issues within the pure world evolve in area and time—machine studying fashions can produce dependable outcomes even when they’re supplied with restricted information.
Their outcomes, reported within the Proceedings of the Nationwide Academy of Sciences, may very well be helpful for developing extra time- and cost-efficient machine studying fashions for functions corresponding to engineering and local weather modeling.
Most machine studying fashions require giant quantities of coaching information earlier than they’ll start returning correct outcomes. Historically, a human will annotate a big quantity of knowledge—corresponding to a set of photographs, for instance—to coach the mannequin.
“Utilizing people to coach machine studying fashions is efficient, but it surely’s additionally time-consuming and costly,” stated first creator Dr. Nicolas Boullé, from the Isaac Newton Institute for Mathematical Sciences. “We’re to know precisely how little information we really need to coach these fashions and nonetheless get dependable outcomes.”
Different researchers have been capable of prepare machine studying fashions with a small quantity of knowledge and get wonderful outcomes, however how this was achieved has not been well-explained. For his or her examine, Boullé and his co-authors, Diana Halikias and Alex Townsend from Cornell College, centered on partial differential equations (PDEs).
“PDEs are just like the constructing blocks of physics: they can assist clarify the bodily legal guidelines of nature, corresponding to how the regular state is held in a melting block of ice,” stated Boullé, who’s an INI-Simons Basis Postdoctoral Fellow. “Since they’re comparatively easy fashions, we would be capable to use them to make some generalizations about why these AI strategies have been so profitable in physics.”
The researchers discovered that PDEs that mannequin diffusion have a construction that’s helpful for designing AI fashions. “Utilizing a easy mannequin, you would possibly be capable to implement a few of the physics that you just already know into the coaching information set to get higher accuracy and efficiency,” stated Boullé.
The researchers constructed an environment friendly algorithm for predicting the options of PDEs below totally different situations by exploiting the quick and long-range interactions occurring. This allowed them to construct some mathematical ensures into the mannequin and decide precisely how a lot coaching information was required to finish up with a sturdy mannequin.
“It relies on the sphere, however for physics, we discovered which you could really do lots with a really restricted quantity of knowledge,” stated Boullé. “It is shocking how little information it’s worthwhile to find yourself with a dependable mannequin. Because of the arithmetic of those equations, we are able to exploit their construction to make the fashions extra environment friendly.”
The researchers say that their strategies will enable information scientists to open the ‘black field’ of many machine studying fashions and design new ones that may be interpreted by people, though future analysis remains to be wanted.
“We have to be sure that fashions are studying the appropriate issues, however machine studying for physics is an thrilling area—there are many fascinating math[s] and physics questions that AI can assist us reply,” stated Boullé.
Extra data:
Nicolas Boullé et al, Elliptic PDE studying is provably data-efficient, Proceedings of the Nationwide Academy of Sciences (2023). DOI: 10.1073/pnas.2303904120
College of Cambridge
Quotation:
Machine studying fashions can produce dependable outcomes even with restricted coaching information (2023, September 19)
retrieved 20 September 2023
from
This doc is topic to copyright. Aside from any truthful dealing for the aim of personal examine or analysis, no
half could also be reproduced with out the written permission. The content material is supplied for data functions solely.