Mana.bio is reimagining how we deliver RNA and gene therapies. Founded by a team of biotech and AI veterans, the startup has built an integrated wet-lab and machine learning platform to design lipid nanoparticles that deliver nucleic acid payloads with precision. Their mission: make drug delivery programmable, predictable, and faster.
1. What inspired the creation of Mana.bio, and what problem were you aiming to solve?
Yogev Debbi: RNA medicines hold enormous potential for the future of therapeutics but, effective, tissue-specific delivery remains a challenge. Development of lipid nanoparticles (LNPs), the primary delivery vehicle, has historically relied heavily on trial and error and empirical testing. Mana’s founding team of repeat entrepreneurs and highly regarded experts in the field of drug delivery, machine learning and software development, are on a mission to make drug delivery programmable, predictable, and faster by building a platform that uses artificial intelligence (AI) to streamline the LNP discovery and optimization process. Our goal is to develop a powerful platform that generates novel delivery solutions to safely and efficiently deliver the right payload to the right tissue, and unlock the full potential of RNA and gene therapies.
2. How does Mana.bio’s AI-driven approach enhance drug delivery for nucleic acid therapeutics and vaccines?
Yogev Debbi: Mana’s platform uses public datasets and proprietary, high-throughput in-house experimental data to train ML models that predict how a specific LNP will perform — in terms of physicochemical properties, potency, specificity, and safety. This offers the potential to accelerate requisite timelines and reduce the cost of developing novel LNP for bespoke applications. In one recent example, our iterative AI modeling cycles improved the in-vivo potency of a novel lung-targeted LNP by 100-fold in just three months. We also recently integrated both predictive potency and safety modeling into one platform to mitigate key safety determinants like immunogenicity and hepatotoxicity without sacrificing efficacy. These synergies can meaningfully reduce the time and cost it takes to bring a new therapy to patients.
3. What were the biggest challenges in developing AI-powered lipid nanoparticle (LNP) formulations?
Yogev Debbi: One of the hardest, but most important, steps was building a truly integrated wet-lab and AI workflow. This is something that no other LNP company has been able to achieve. LNPs are defined, yet complex systems, and making sense of their behavior requires high-quality, consistent data across biology, chemistry, and delivery performance. Mana has built proprietary systems to collect, clean, and interpret that data in real time, and feed it back into our models for continuous improvement. It took time, experimentation, and a lot of interdisciplinary collaboration, but it’s what powers our ability to quickly and reliably advance from design to optimized lead candidate.
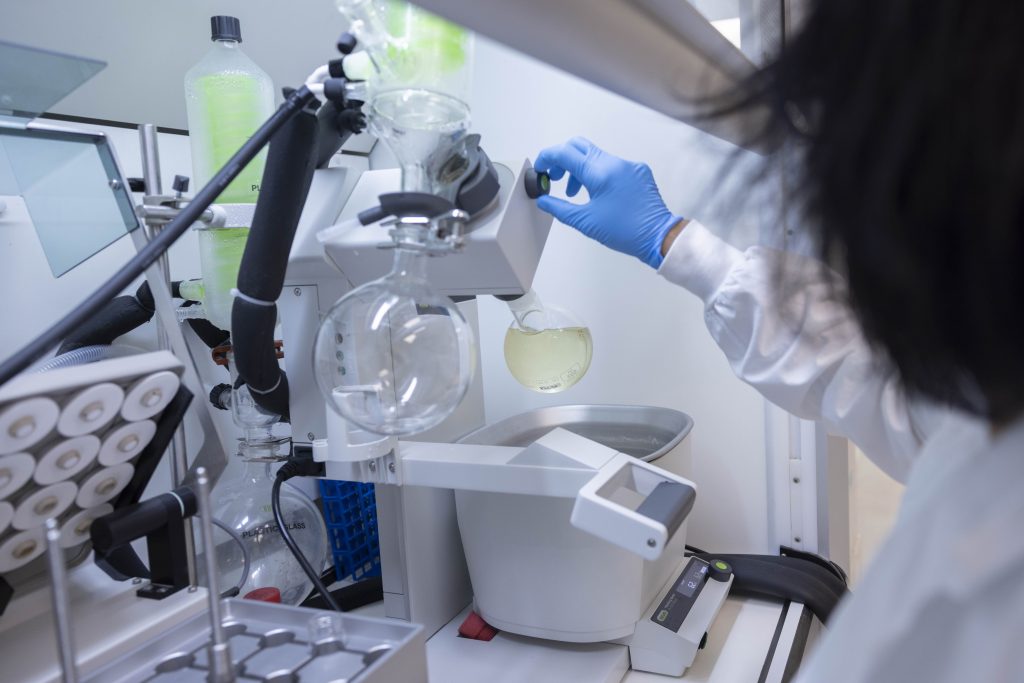
Credit: Tomer Appelbaum – TheMarker
4. How has your experience in securing funding and partnerships influenced Mana.bio’s growth trajectory?
Yogev Debbi: Securing strong early investment was a major inflection point for Mana.bio. Our $19.5M seed round — backed by Andreessen Horowitz Bio + Health, Base4 Capital, NFX, LionBird, and Technion — gave us the resources to move quickly and build with real intention. These partners brought more than capital; they brought deep expertise in biotech and AI, and a shared belief in the future of programmable drug delivery. Their early conviction validated our vision and helped us scale our wet-lab and AI capabilities in a meaningful way.
Working with our partners has really helped shape our direction in two meaningful ways. First, validating our delivery results in the hands of our biopharma and hospital research partners has been an affirming endorsement of the power of Mana’s platform. It’s reassuring to know that our AI-driven approach holds up in real-world settings. Second, these collaborations have helped us stay focused on what matters most. One of Mana’s key near-term corporate goals is to provide partners with an LNP delivery solution. Our partners bring a clear sense of purpose and urgency, and their belief in what we’re building continues to motivate and guide us as we move forward.
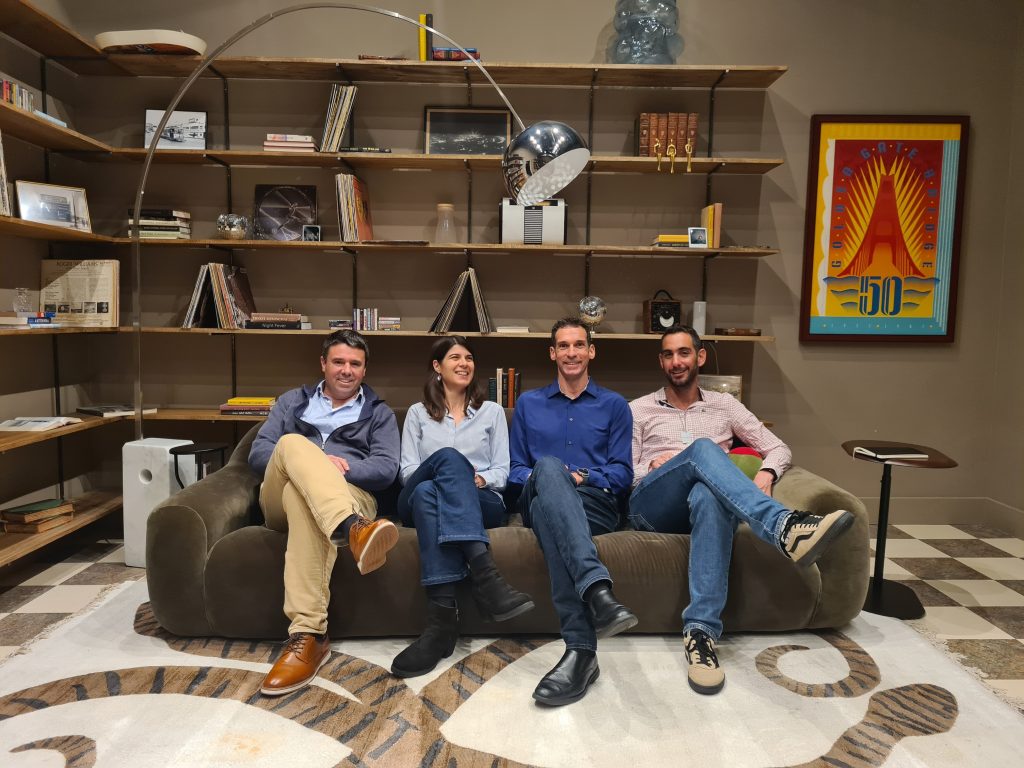
5. Can you explain how Mana.bio’s AI platform designs and optimizes LNP formulations?
Yogev Debbi: At the heart of our platform is an iterative “design-build-test-learn” loop powered by machine learning. We combine high-throughput lab experimentation with curated public datasets to train ML models that predict how specific LNP formulations will perform — not just in vitro, but across species, including mice and non-human primates. These models guide the designof novel lipids and particle compositions based on a specific goal like specific tissue targeting. Mana can then buildrecommended LNP that are prioritized on criteria such as predicted activity, novelty, and scalability, and empirically testtheir performance in the lab. The data generated is then fed back into Mana’s AI/ML engine to continuously learnfrom every data point. The result is a truly programmable approach to drug delivery that can be customized for different payloads, tissues, and therapeutic needs.
6. What makes your machine learning-based drug delivery system unique compared to traditional methods?
Yogev Debbi: Traditionally, LNP discovery has been driven by rational design and experimental trial-and-error. This artisanal process is slow and labor-intensive, and relies on niche know-how of highly-talented chemists. High-throughput barcoding platforms have opened opportunities to simultaneously evaluate panels of LNP compositions, but these highly technical and expensive platforms often encounter challenges when scaling candidates to therapeutic doses. Both approaches also lack the ability to continuously learn from each data point, and retroactive learning on existing datasets is nearly impossible without integrated wet-lab + AI workflow. Our system disrupts these approaches by embedding AI into every step of the discovery process. It’s not just faster — it’s smarter, safer, and more adaptable.
7. How do you see AI and nanotechnology reshaping the future of gene therapy and precision medicine?
Yogev Debbi: AI opens the possibility to significantly accelerate the drug development process. Instead of relying on trial and error, AI can digest all relevant public and proprietary data to continuously learn from every single experiment. This systematic analysis of a vast chemical and biological universe is not possible without AI. We can then integrate several predicted considerations such as stability, potency and safety to develop a delivery vehicle for an intended purpose. Moreover, by combining AI with nanotechnology, we’re opening the door to treatments that are more precise, more effective, and more accessible — across a wide range of conditions, maximizing patient impact.
8. What are the main benefits for biotech companies and pharmaceutical firms integrating Mana.bio’s platform?
Yogev Debbi: For our partners, it’s about speed, reliability, and de-risking development. Instead of investing years into empirical formulation testing, companies can use Mana’s platform to engineer targeted delivery systems in just months, which are tailored to their specific needs (i.e. payloads, cell types and more). That means faster transitions from discovery to preclinical — and fewer surprises along the way. Additionally, we’ve built in a proprietary tool to evaluate novelty from IP perspective to ensure there is market potential for any lead candidate LNP. Whether you’re a large pharma company with a complex pipeline or a biotech startup with a single high-stakes asset, integrating our platform helps you move smarter and faster toward the clinic, while saving requisite investment of building in-house delivery capabilities.
9. What have been the biggest challenges in scaling Mana.bio, and how did you overcome them?
Yogev Debbi: It’s all about people. We are grateful to have such an amazing team of talented, experts and motivated individuals who truly collaborate as a team. One of the early biggest challenges was bringing together people from such different backgrounds — from biology and chemistry to machine learning and software. We had to build a shared language and workflow that allowed everyone to effectively collaborate, appreciate each other’s perspective, and bridge knowledge gaps to create a well-oiled machine. Everyone on the team is here because they believe in the impact this work can have, and that’s been our anchor through it all.
10. AI-powered drug development often faces ethical and regulatory concerns—how does Mana.bio ensure compliance and patient safety?
Yogev Debbi: We take this responsibility seriously. From the beginning, we’ve designed our platform to prioritize transparency, reproducibility, and biological relevance. Our AI models are trained on high-quality, experimentally validated data, and every prediction is followed by rigorous lab testing. We also work closely with scientific advisors, regulatory consultants, and potential partners to ensure we’re aligning with evolving standards. Ultimately, our goal is not just to move fast — it’s to move responsibly, with patient safety at the center of everything we do.
11. What does success look like for you and Mana.bio in the next five years?
Yogev Debbi: For us, success means seeing therapies powered by our platform reach the clinic — and ultimately, patients. We want to become the go-to partner for companies developing nucleic acid-based therapies, whether for rare diseases, oncology, or gene editing. On a deeper level, success means proving that drug delivery can be programmable, predictable, and personalized, showing that an AI-native approach can shift what’s possible in medicine.
12. What does a typical day look like for your team, and how do you stay motivated in such a cutting-edge field?
Yogev Debbi: Our mornings start with a daily “standup” meeting (like a typical tech company) – aligning on previous day’s progress and on what is expected today. This way we maintain communication, alignment, and visibility across all issues or changes in plans. In a similar way, we also do a weekly kick off meeting to align at the beginning of the week and another at the end of the week, so everyone can demo their progress and discuss different items. During any given day the team members can track everyone’s progress by staring at the various dashboards around the office – from data points generated in the lab, to models predictions accuracy and more projects tracked. Lastly to emphasize, the focus every day can be different — and that’s part of what makes this so exciting. Some days are more in data analysis, others are more in the lab. We’re constantly learning from each other across disciplines. What keeps us motivated is the shared sense that we’re working on something bigger than ourselves and that we’re disrupting the way that drug development is done. We’re not just solving a technical challenge; we’re helping build the foundation for the next generation of medicine. That purpose shows up in the way we work, the way we collaborate, and the energy we bring to the mission every day.
Editor’s Note
Yogev Debbi and the Mana.bio team are tackling one of medicine’s most overlooked bottlenecks—targeted delivery. This interview highlights how AI can transform the speed and safety of drug development, unlocking new frontiers in gene therapy, RNA medicine, and personalized healthcare.
Discover more interviews with AI founders on the AIPressRoom Interviews page.