Moving data slows things down everywhere. Data is what artificial intelligence needs. Scalytics transforms that by allowing artificial intelligence to operate where the data is already housed. This eliminates the necessity for compliance concerns and ETL delays. Co-founder of Scalytics Alexander Alten discusses how his work in corporate data helped the business develop. He also discusses the challenges of distributing artificial intelligence and clarifies why the future of AI is faster, safer, and built for real-world data.
1. What inspired you to create Scalytics, and how did your prior experiences shape the product’s vision?
Alexander Alten: We were engineers at Cloudera (Mirko and I), and Jorge was a Senior Researcher who started to research in-situ cross-platform data analytics as he foresaw that multiple data platforms would dominate the market in the future. I then left for Allianz, a European insurance company, and witnessed the problems of working with data stored in hundreds of data silos. Shortly after, I joined Evariant, a healthcare company in the US. I saw that something better needs to be done as we have; Hadoop was widely used, and Spark came – but it’s all the same; you need to move your data to them. That didn’t make sense these days, and for sure it doesn’t make any sense now, especially when AI starts to go mainstream. In 2018, I met Jorge again, and so it all started; Scalytics was born in 2022.
2. What challenges did you face when transitioning from a centralized data model to decentralized federated learning?
Alexander Alten: Ideology. Data marts and data silos have been in information technology since databases were invented. They are called data marts, data warehouses, data lakes or whatever, but the principle is the same: a centralized store of data, and when you will call it, value.
3. How does Scalytics differentiate itself in the crowded AI and ML platform landscape?
Alexander Alten: We managed to build a federated data processing model around data silos, which allows data analytics on data that is typically not movable. Now, this extends to AI and, of course, agentic solutions like RAG. With Scalytics Connect, you are able to add data sources dynamically to your AI or analytics stack without replatforming your current solution. Right now, with our MCP implementation, users are able to leverage Kafka as a streaming platform and train AI locally with dynamic data. From energy to healthcare, live data is directly accessible without ETL or data lakes.
4. Scalytics promotes transparency and scalability in AI development. Can you share a real-world use case where this approach made a significant impact?
Alexander Alten: Energy – right now, AI needs much more energy to power the infrastructure. That causes problems in grid stability, distribution and management. We are able to build real-life digital twins based on near real-time data from transformers and distribution facilities and predict energy consumption. In healthcare, Scalytics helps to accelerate research by combining PHI or HIPAA-related data without exposing them. They stay on their origins; only AI or ML models use them to calculate patterns.
5. How does the Scalytics platform help organizations overcome regulatory challenges like GDPR and HIPAA while maintaining operational efficiency?
Alexander Alten: As we use in-situ data processing, the raw data stays at the original store. We don’t ETL it out into a data lake or data mart, and we introduce maximal control over the use of the restricted data. This solves the problem of regulated and highly protected data sets—we provide the capability to gain insights and use the data without tampering with regulations.
6. What role does Scalytics’ federated learning play in improving collaboration across decentralized teams and organizations?
Alexander Alten: Scalytics Connect enables secure collaboration by allowing organizations to train machine learning models without sharing raw data. For instance, hospitals can collectively develop disease detection models while keeping patient data private, fostering innovation while complying with regulations like GDPR. In finance, banks can identify global fraud patterns by collaboratively training models across regions without pooling sensitive data. This approach enhances decision-making by leveraging diverse insights while ensuring security. As Scalytics Connect is built on top of Apache Wayang, it also provides seamless cross-platform integration, removing technical barriers to collaboration and driving faster, more impactful innovation.
7. Your platform eliminates traditional ETL bottlenecks. How does this improve time-to-market for AI projects?
Alexander Alten: In a green field approach we are able to deliver the first result in hours. For example, you’d have Databricks, Snowflake, Postgres, and maybe a MongoDB, and you want to analyze how the quality of your products improved, or not improved, based on user feedback, detect patterns to develop optimizations, eventually using Databricks ML for that. Right now, you’d need a lot of data moving, ETL and preparation, de-duplication, batch processing etc. All these cost time, resources, and money. With Scalytics, the platforms would be virtually connected, and you can run your AI on Databricks, using the data you have already there and even adding more data sources without rebuilding the stack or implementing more ETL. The data will be processed in situ, meaning in Snowflake, Postgres, or MongoDB, using the resources there. Your time-to-market reduces drastically; you focus on your idea and not building a data infra for every idea or project you have.
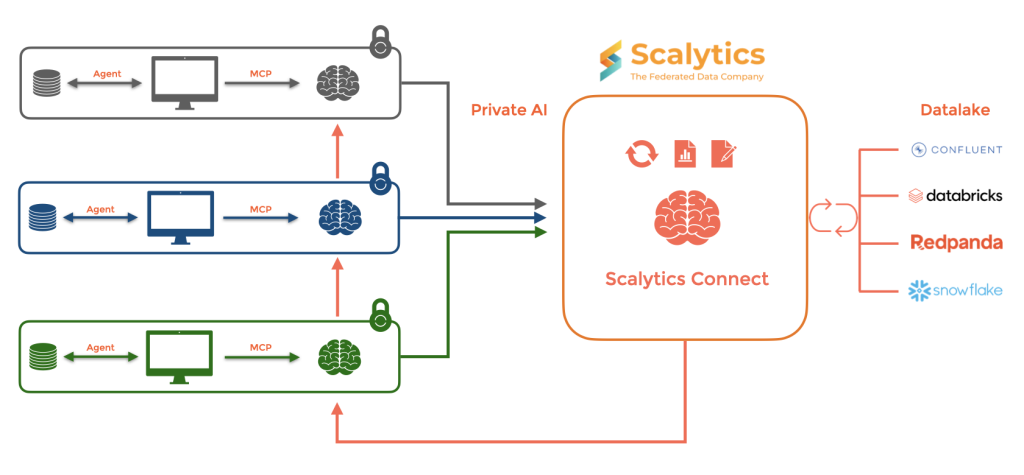
8. Looking ahead, how do you see Scalytics shaping the future of AI development and adoption across industries?
Alexander Alten: We’re on the verge of data decentralization. We know that we use all the data to train the LLMs we have right now, but we never use the data organizations and industries create. But those are the ones who bring value; those are the ones who allow me to innovate and be faster in product development, drug discovery, and research. Shift-left architectures come into play again. Data is generated on the edge, and AI lives on the edge. There is the maximum value, and we enable it.
9. What has been the most rewarding and challenging part of your journey in building Scalytics?
Alexander Alten: As we ran the first distributed code, we used the TPC-H benchmark. It was in the early days, and it proved that our research worked.
10. As a founder, how do you balance the technical demands of innovation with the strategic goals of scaling the business?
Alexander Alten: We concentrate on customer needs and work our way backward. It’s not technology that solves current problems; it’s the way you solve them. We’re bootstrapped, and we listen carefully to what design partners tell us. Make your tech simple to use, and do the hard work behind the scenes.
11. What does success mean to you, and what are your aspirations for Scalytics in the coming years?
Alexander Alten: We want to leave something useful behind. We want to enable AI for every enterprise in the world, give them the freedom to choose their tool of choice and remove technology lock-ins. Scalytics is a needed bridge between technology worlds.
12. What does a typical day look like for you as the CEO of Scalytics?
Alexander Alten: I start at eight, checking email/messaging, checking team communication, and meeting until noon. Then, I go out with my dog; he’s old and can’t walk fast. Afterward, I concentrate on market requirements, listen to my co-founders, and talk with partners.
Editor’s Note:
Alexander Alten believes that artificial intelligence is about better use of data, not about data movement. Scalytics Connect allows businesses to apply artificial intelligence to data about finances, healthcare, and energy without exposing it or relocating it. Scalytics removes ETL bottlenecks and integrates with current systems, therefore preparing AI to grow and making it safer. Explore more insights from AI founders in our Interviews section.